Journal of Energy Markets
ISSN:
1756-3607 (print)
1756-3615 (online)
Editor-in-chief: Derek W. Bunn

Electricity market prices for day-ahead ancillary services and energy: Texas
Jay Zarnikau, Chi Keung Woo, Shuangshuang Zhu, Ross Baldick, Chen-Hao Tsai and Jingwei Meng
Need to know
- We explore price formation in the day-ahead markets for electrical energy and ancillary services operated by the Electric Reliability Council of Texas.
- The amounts of ancillary services required by the system operator and procured through the day-ahead market have been remarkably stable and have even very slightly declined over the 2011 to 2017 period, despite a large expansion in Texas’ wind generation.
- The prices of ancillary services have also slightly declined, due to economic merit order effects induced by wind generation, changes in natural gas prices, the participation of interruptible loads in the market for responsive reserves, and other factors.
- We find that the markets for responsive reserves and regulation-up are efficient, in the sense that a one-unit increase in day-ahead energy prices tends to increase the prices of those two operating reserves by roughly one unit.
Abstract
Applying a regression-based approach to a large sample of hourly observations for the seven-year period of January 1, 2011 to December 30, 2017, we explore determinants of day-ahead market (DAM) prices for ancillary services (ASs) and energy in the Electric Reliability Council of Texas (ERCOT). For each GW increase in responsive reserve (RRS) or nonspinning reserve (NSRS) procurement quantities, we estimate price increases of about US$3.47 per MW per hour and US$5.63 per MW per hour, respectively; while the cost of an additional 1 GW of regulation up (REGUP) and regulation down (REGDN) is a much higher US$17 per MW per hour and US$31 per MW per hour, respectively. A US$1/MWh increase in the DAM energy price tends to increase RRS and REGUP prices by nearly US$1 per MW per hour, about twice the estimated impact for NSRS that is not necessarily online when selected. The participation of interruptible loads to provide RRSs reduces the RRS price, and this effect increased nearly six-fold from the first to the second half of our sample time period. An increase in wind generation tends to decrease AS prices because it reduces the DAM energy price via the merit-order effect. Hence, Texas’s wind generation expansion has not raised ERCOT’s AS prices in our sample period, which is characterized by a stable AS requirement and declining natural gas prices. Going forward, however, Texas could face AS cost escalation due to the high REGUP and REGDN prices, should ERCOT’s requirement and procurement of those services increase due to rising renewable production.
Introduction
1 Introduction
In a network designed to satisfy the electricity needs of energy consumers, supply and demand must balance in real time to maintain system reliability and frequency. This poses a challenge to system operators due to random fluctuations in system load; unexpected changes in generation output (eg, intermittent renewable energy sources); errors in forecasting demand and generation; unexpected mechanical failures of generation or transmission assets; and limited ability to economically store electricity in a network dominated by thermal resources. As a result, the procurement of various ancillary services (ASs) by system operators is necessary to resolve the inevitable fluctuating demand–supply imbalances that occur in networks between updates of the economic dispatch set-points of generators (Stoft 2002).11 1 The Federal Energy Regulatory Commission (FERC) defines ASs as services “necessary to support the transmission of electric power from seller to purchaser given the obligations of control areas and transmitting utilities within those control areas to maintain reliable operations of the interconnected transmission system”. See FERC Orders 888, 889 and 2000.
As competitive wholesale electricity markets have evolved, attention has focused on (a) establishing performance standards for AS resources, (b) the design of competitive markets for the procurement of ASs and (c) determining the optimal levels of AS requirements (Oren 2002). Increasing dependence on randomly intermittent and as-available renewable resources such as solar and wind may increase a network’s AS needs, sparking investigations into how such needs may be met in the future (GE Energy 2008; ERCOT 2013; Exeter Associates and GE Energy 2012; National Renewable Energy Laboratory 2011a,b; Andrade et al 2017).
The primary objective of this paper is to empirically characterize, via a regression-based approach, the AS prices data-generating process (DGP) in a competitive market. This characterization is useful and relevant for the following reasons. First, there has been little attention devoted to the market price behavior of ASs, notwithstanding a review of historical prices in US markets by Argonne National Laboratory (2016) and periodic analyses performed by the independent market monitor of the Electric Reliability Council of Texas (ERCOT) (Potomac Economics 2018). Second, a network’s AS costs are nontrivial, at least in the aggregate, and ultimately borne by consumers, due to load-serving entities’ pass-through of such costs that move with the AS prices.22 2 Based on Argonne National Laboratory (2016, p. 34), the annual market revenue for regulation services added across the California (CAISO), Midwest (MISO), PJM and Southwest Power Pool (SPP) markets in 2014 was about USD383 million. For spinning or responsive reserves, the total was USD139 million in that year for those markets. The annual market revenue for nonspinning reserves in those markets in 2014 totaled USD36 million. Finally, little is known regarding how AS prices may vary with a network’s dependence on renewable generation, unlike the well-documented merit-order effects of renewable generation on day-ahead market (DAM) and real-time market (RTM) energy prices (see, for example, Woo et al (2011, 2015, 2016a, 2017, 2018) and Zarnikau et al (2016), and the extensive references therein).
We exploit the interdependence of ASs and energy markets to develop a model of price regressions in order to analyze hourly data obtained from ERCOT. ERCOT serves 85% of the electrical needs of the largest electricity-consuming state in the United States and accounts for over 9% of the nation’s total electricity generation.33 3 Generation in ERCOT was 375 890 GWh in 2017, as reported to the North American Electric Reliability Corporation (https://bit.ly/2aQGG6C). Total US generation at utility-scale facilities was 4 014 804 GWh in that year, according to the US Department of Energy’s Energy Information Administration (https://bit.ly/JI2kY3). Moreover, ERCOT is repeatedly cited as North America’s most successful attempt to introduce competition in both generation and retail segments of the power industry (Distributed Energy Financial Group 2015). Finally, Texas has greatly increased its dependence on intermittent renewable energy generation over the time period studied here.
Our comprehensive analysis of ERCOT’s DAM energy and AS prices finds the following.
- •
ERCOT’s DAM energy price moves with such fundamental drivers as natural gas price, system load, nuclear generation, wind generation and AS requirements. In particular, it declines with nuclear and wind generation but increases with the other drivers.
- •
Because of market interdependence between ASs and energy, ERCOT’s AS prices increase with the DAM energy price. They also increase with ERCOT’s AS demands as measured by the procurement quantities but decrease with ERCOT’s AS supplies as measured by the offer quantities.
- •
A USD1/MWh increase in the DAM energy price tends to increase the responsive reserve (RRS) and regulation up (REGUP) prices by nearly USD1 per MW per hour, indicating market efficiency that results from the arbitrage behavior and co-optimization among generating units that provide RRS and REGUP services and can also supply DAM energy.
- •
A USD1/MWh increase in the DAM energy price tends to raise the nonspinning reserve (NSRS) price by a lesser amount of about USD0.44 per MW per hour, reflecting that providers of this service are not necessarily online and burning fuel, and thus incur lower costs to provide NSRSs.
- •
The per MW per hour price of acquiring additional REGUP and regulation down (REGDN) services is considerably more than that of RRSs or NSRSs, reflecting that few generation and load resources can respond fast enough to system changes to qualify as REGUP and REGDN providers.
- •
The rising share of RRSs provided by load resources tends to reduce the RRS price because ERCOT’s interruptible loads often offer demand reductions at zero cost, thereby raising their likelihood of being awarded.
Taken together, the above findings suggest that large-scale renewable energy development in Texas has not increased ERCOT’s AS prices during our sample period; nor has it increased ERCOT’s AS procurement costs, thanks to ERCOT’s stable AS requirements and procurements. Further, ERCOT’s success in facilitating the participation of load resources in the market for RRSs has proven successful in constraining the market prices of that service, lending support to FERC’s actions to ensure load resources have the opportunity to participate in AS markets in other regions of the United States. Going forward, however, there is a potential risk for AS cost escalation in Texas because of the high per MW cost for additional REGUP and REGDN procurement, which may rise in response to further expansion of wind energy development in the state.
This paper makes the following contributions to the literature on electricity market price behavior. First, to the best of our knowledge, it is the first detailed regression analysis of AS prices in ERCOT’s DAM environment. Second, the analysis is comprehensive, encompassing all of ERCOT’s day-ahead products: energy, RRS, NRRS, REGUP and REGDN. Finally, its parsimonious and easy-to-understand regression specification is general and equally applicable to other regional grids with data availability similar to ERCOT’s (eg, California, New York, New England and PJM).
This paper proceeds as follows. Section 2 provides an overview of the ERCOT market and explains the determination of AS requirements, obligations, and procurement. It also describes our data sample and proposes our regression specification. Section 3 presents the regression results. Section 4 discusses these results. Section 5 contains our conclusions.
2 Materials and methods
2.1 Why ERCOT?
ERCOT presents an interesting setting for analyzing AS market price behavior because formal markets for both energy and ASs have been established to foster competition, these markets are of significant size, and Texas leads the nation in electricity generation from wind farms. Increased generation from intermittent renewable energy sources, such as wind, has prompted concerns that the state’s need for ASs may increase in the future, perhaps raising the cost of maintaining adequate ASs. Consequently, an examination of how growing wind generation has affected market prices of ASs in Texas can inform other markets with large-scale renewable energy development.
ERCOT has undergone gradual restructuring since the mid-1990s (Zarnikau 2005). Legislation enacted in 1995 required the Public Utility Commission of Texas to establish rules to foster wholesale competition and create an independent system operator (ISO) to ensure nondiscriminatory access to the transmission network. Sweeping reforms were introduced through Senate Bill 7 in 1999, allowing customers of the investor-owned utilities within ERCOT to choose among competitive retail electric providers (REPs) for a retail supply of electricity beginning on January 1, 2002. Senate Bill 7 also enhanced the ISO’s centralized control over the wholesale market, replacing ten control centers formerly operated by various utilities. This led to the establishment of a formal DAM for ASs and an RTM for balancing energy.
An important structural change to the ERCOT market was completed on December 1, 2010: the introduction of a nodal market structure (Zarnikau et al 2014a). The DAM was expanded from merely a market for the procurement of operating reserves to also include energy. Similar to the DAM energy markets elsewhere (eg, PJM, New York, New England and California), ERCOT’s DAM matches day-ahead forward energy bids and offers (Zarnikau et al 2014b). Hourly market-clearing DAM energy prices used to settle DAM transactions result from a least-cost dispatch that co-optimizes energy generation with AS and congestion revenue rights.
Supply offers and bids begin at 06:00 and end at 10:00 on day for ERCOT’s day-ahead price determination. DAM is normally executed between 10:00 and 13:30, and prices for the following day are set around 14:00, though prices may be updated by 18:00 after any needed reliability unit commitment activities are completed.44 4 See ERCOT training module, Module 2: Day-Ahead Operations, available from the ERCOT website. As actual wind generation and total energy demand on day are not known on day , the DAM energy prices for energy and ASs depend, in part, on forecasts of wind generation and load made on day in the 06:00–10:00 window.
There is presently no “capacity market” in ERCOT to maintain a target reserve margin. Consequently, ERCOT relies on market forces to achieve reliability and resource adequacy. To encourage investment in generation capacity, the offer cap on wholesale market prices was raised to USD3000/MWh at the start of the nodal market on December 1, 2010. The cap was further increased to USD4500/MWh, effective August 2012; USD5000/MWh on June 1, 2013; USD7000/MWh on June 1, 2014; and USD9000/MWh on June 1, 2015. ERCOT’s DAM energy prices, however, have seldom reached this cap since the nodal market’s establishment.
Texas is home to the most wind generation in the United States, thanks to its enormous resource potential; establishment of policy targets; a system of tradable renewable energy credits (RECs) and federal tax credits; and ERCOT’s favorable market rules (Zarnikau 2011). The completion of Competitive Renewable Energy Zone transmission in January 2014 resulted in 3600 circuit-miles of 345 kV transmission line to increase export capability in order to accommodate approximately 11 GW of additional power for a total of 18.5 GW of renewable capacity, further unlocking the wind potential in West Texas. The intermittent nature of wind generation resources has presented operational challenges to ERCOT, and the establishment of new market rules was necessary in order to accommodate this nondispatchable intermittent resource. In addition, ERCOT has been improving its ability to accurately forecast wind generation output.
2.2 Ancillary services in ERCOT
Product | Description |
---|---|
Regulation-up (REGUP) |
Must immediately increase generation output (or reduce demand, if a load resource) in response to automated signals to balance real-time demand and resources |
Regulation-down (REGDN) |
Must immediately decrease generation output (or increase demand, if a load resource) in response to automated signals to balance real-time demand and resources |
Responsive reserve (RRS) |
Each resource providing RRS must be online, frequency responsive based on governor action and fully responsive to any automated or verbal dispatch instructions from ERCOT within ten minutes A load resource providing RRS must immediately respond when system frequency drops below 59.7 Hz, and it must be able to maintain the scheduled level of deployment for the period of the service commitment |
Nonspinning reserve (NSRS) |
Each resource providing NSRS must be capable of being synchronized and ramped to its schedule for NSRSs within thirty minutes Nonspin may only be provided from capacity that is not fulfilling any other energy or capacity commitment |
As summarized in Table 1, ERCOT presently procures four types of system-wide operating reserves: REGUP, REGDN, RRS and NSRS, similar to the system operators elsewhere (eg, California, New York, New England and PJM).
REGUP and REGDN capacities balance small fluctuations in supply and demand in real time to maintain system frequency close to 60 Hz. These services are primarily provided by generators with automatic generation control (AGC) or equivalent. Resources providing regulation services must respond every four seconds, either by increasing or decreasing output to address gaps between supply and demand, and must comply with ERCOT instructions within five minutes.
Similar to the spinning reserves maintained in many other electricity markets, an RRS restores the balance between generation and load after the sudden forced outage of a major generator or transmission line or some other major shock to the electrical system (eg, an unexpectedly large drop of wind speed in West Texas, where most of the state’s wind farms reside). The dispatcher must therefore have an RRS available to prevent an unacceptable drop in system frequency.
RRS can be provided by unloaded generation resources that are online but not generating at full capacity, and can therefore increase their output quickly to provide additional capacity to the system. As with generation resources providing REGUP and REGDN, generators providing RRSs must be responsive to automated ERCOT instructions, via AGC that translates instructions into the adjustment of the prime mover and electrical output. In addition, properly qualified load resources can provide RRSs. Nearly half of ERCOT’s RRS requirements are met by load resources equipped with under-frequency relays that instantaneously curtail load when frequency drops to 59.7 Hz. Resources providing this service must also be able to respond to verbal dispatch instructions. Requirements for RRSs are calculated in four-hour blocks on the basis of forecasted load and wind patterns. During the sample period, load resources were permitted to provide no more than 50% of RRS requirements, thus ensuring sufficient generation resources to maintain adequate inertia on the system; although the limit was subsequently raised to 60%, effective May 31, 2018.55 5 See ERCOT “Percent change in load-provided responsive reserve” on the ERCOT website.
NSRS ensures sufficient capacity to cover large forecast errors or replace a deployed RRS. While resources providing RRSs must increase output in compliance with ERCOT instructions within ten minutes, resources providing NSRSs must comply within thirty minutes. NSRSs may be provided by generation units that are offline as long as they are able to start up and increase their output to the target level within a predefined period of time, usually ten to thirty minutes. Small amounts of NSRS have historically been provided by load resources.


ERCOT establishes hourly requirements for each operating reserve depicted in Figure 1. Requirements changed greatly when the nodal market was introduced in December 1, 2010 and generation prices started to be calculated every five minutes (at most), rather than every fifteen minutes. In particular, the need for REGUP and REGDN was reduced accordingly, mirroring the shorter time between a security-constrained economic dispatch solution and the start of an operating period, which is presumed to decrease short-term forecasting errors. In light of the confounding structural changes that accompanied the introduction of the nodal market, the AS prices and procurements during the zonal market period will not be considered in this analysis.
For REGUP, requirements can change from hour to hour, depending on historical wind generation and other factors. At the start of the nodal market, regulation requirements were set based on an examination of changes in net load (demand minus wind generation) the same month of the previous year and the past thirty days prior to the time of a study; refinements were applied in February 2011.66 6 See 2010–2011 Ancillary Service Methodology, ERCOT Board of Directors, July 20, 2010. Considerable changes to the method used to set minimum regulation requirements were made on January 1, 2016, which resulted in lower requirements.77 7 See Item 9: 2016 Methodology for Determining Minimum Ancillary Service Requirements, ERCOT Board of Directors, December 8, 2015. A small change to the relationship between wind generation and regulation requirements was implemented at the beginning of 2014.88 8 See Item 8: Proposed Changes for the 2014 Methodology for Determining Minimum Ancillary Service Requirements, ERCOT Board of Directors, December 2013. The discussion for REGDN is entirely analogous and hence omitted for brevity.
RRS requirements change less often than other ASs. RRS requirements were historically nearly always 2300 MW, but on April 1, 2012 they were raised to 2800 MW,99 9 See Item 9: 2012 Ancillary Services Methodology Recommendation, Memo from John Dumas to ERCOT Board of Directors, February 21, 2012. and since June 1, 2015 they have been based on variable hourly needs.
While initially set at a fixed hourly amount, NSRS requirements were based on the largest unit planned to be in operation for periods of projected higher risk through most of the zonal market period. This was changed to 1500 MW per hour beginning April 1, 2012.1010 10 Ibid. Subsequently, the system requirement for NSRS was determined by first calculating the ninety-fifth percentile of net load uncertainty from both the previous thirty days and the same month of the previous year.1111 11 Net load is defined as total load minus wind generation, and net load uncertainty is defined as the difference between the realized net load and forecast net load. Solar generation was also included in this definition beginning in January 2017. See Item 9: 2017 Methodology for Determining Minimum Ancillary Service Requirements, ERCOT Board of Directors, December 13, 2016. ERCOT would then subtract the REGUP requirement from this ninety-fifth percentile to obtain the NSRS requirement. In 2016, the formula was altered to remove consideration of net load uncertainty during the previous thirty days, and to instead consider net load uncertainty in the same month of the previous three years.1212 12 See Item 9: 2016 Methodology for Determining Minimum Ancillary Service Requirements, ERCOT Board of Directors, December 8, 2015. During the hours of 07:00 through 22:00 (central time), ERCOT also applies a minimum NSRS requirement equal to the largest single unit in the system. Generally, an improvement in ERCOT’s accuracy in predicting next-day load and wind generation levels reduces ERCOT’s requirements for this service.






Load-serving entities (LSEs) are assigned AS obligations based on their share of the load on the same hour of the previous day. If the LSE served 10% of the load at 14:00–15:00 yesterday, they have an obligation for 10% of each operating reserve AS at 14:00–15:00 today. With advanced notice to ERCOT before the DAM’s price determination, LSEs can self-arrange to meet part or all of their AS obligation. They can use their own generating capacity or interruptible load, or acquire this through a market outside of the DAM. Trades among market participants are common. Figures 2–4 indicate the percentage of AS obligations that are self-arranged for REGUP, RRS and NSRS.1313 13 Per ERCOT Nodal Protocol Section 4.4.7.1.1, a scheduling entity (QSE) may submit a negative self-arranged AS quantity in the DAM, and ERCOT shall procure all negative self-arranged ASs submitted by a QSE.


Many of the larger LSEs that also have generation assets seek to fully hedge (ie, fully self-arrange) and thus avoid their risk exposure to the DAM’s AS price volatilities. The rural electric co-operatives contend that they may encounter federal tax problems if they profit from trading activities unrelated to satisfying the energy needs of their customers.1414 14 See various statements and testimony filed in PUCT Docket No. 31540: Proceeding to Consider Protocols to Implement a Nodal Market in the Electric Reliability Council of Texas Pursuant to PUC Subst. R. 25.501. Consequently, some co-operatives may avoid using the DAM to sell any ASs. During the zonal market period, NSRS was typically fully self-arranged, so that there was no market for this operating reserve. However, following some market changes, this has become unusual.
Whatever portion of an LSE’s obligation is not self-arranged is procured on behalf of the LSE by ERCOT through the DAM.1515 15 In certain ERCOT systems and databases, the amounts procured are referred to as the amount awarded. Certain ASs are not acquired through the DAM (eg, black start and voltage support) and shall be ignored here. These quantities are indicated in Figure 5. The DAM is an hourly market for both energy and ASs, whose prices are determined between 10:00 and 13:30 for the twenty-four hours of the following day. ERCOT bills the LSE for the cost of procuring ASs on the LSE’s behalf at a single market-clearing price of capacity (MCPC) for each AS for each hour.
Generation and load resources supplying ASs and energy are co-optimized in the DAM. Thus, a resource offered to the DAM will end up, if selected or awarded, providing AS or energy, depending on where it has the most value. However, not all resources are qualified to provide all types of AS. A resource that responds slowly will not be selected to provide a service that requires a fast response, such as REGUP.

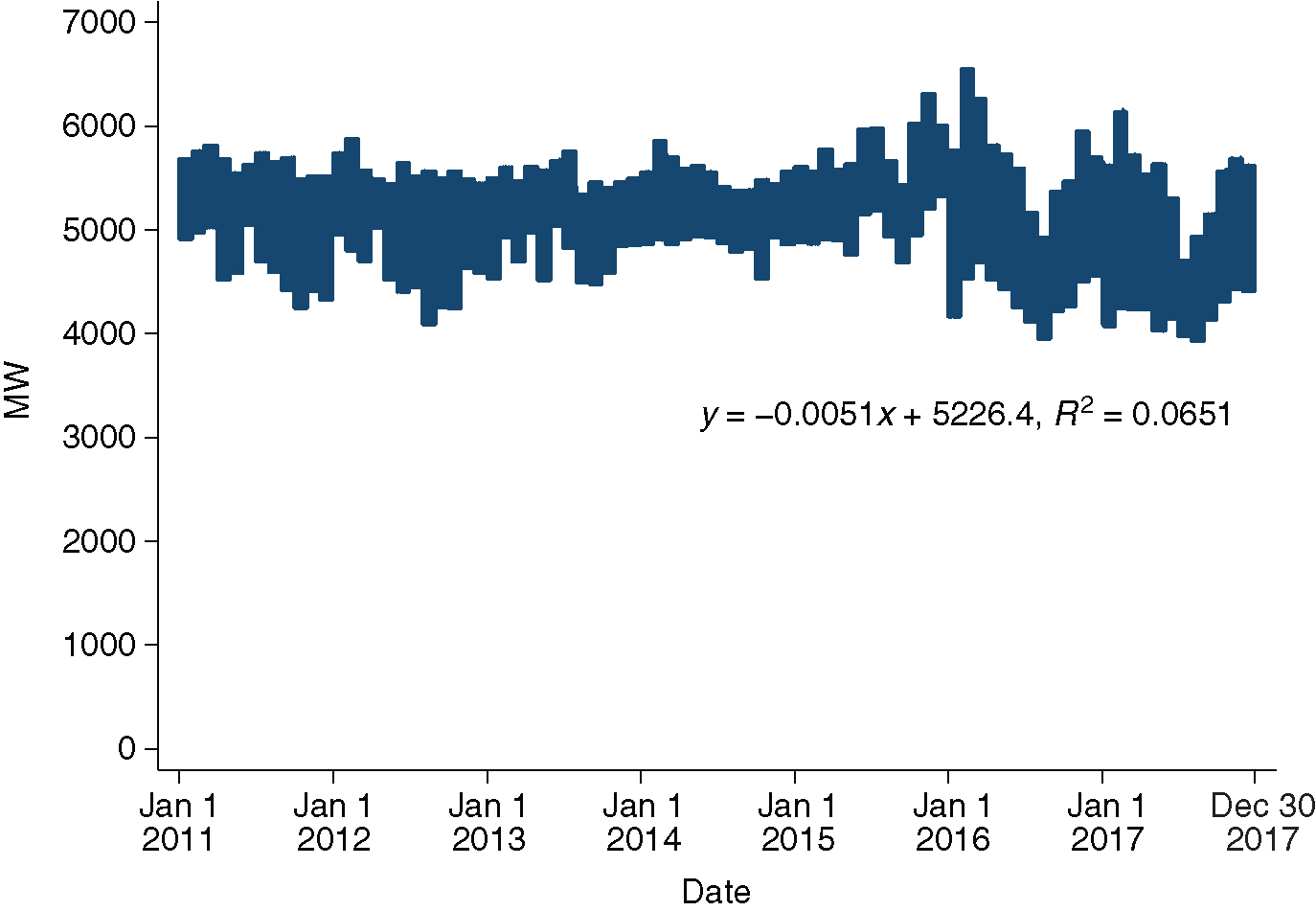
There is often an overabundance of load resources to provide RRSs at USD0/MW in hopes of ensuring their offer is accepted. These resources have negligible deployment costs and count on generators to set the market-clearing price. Formerly, load resources were allowed to offer capacity at negative prices, leading to occasional negative market-clearing prices. Negative-price bidding by load is no longer allowed.1616 16 See the background materials for NPRR 150: Responsive Reserve Service Offer Floor, 2008; and Nodal Protocol Section 4.4.7.2.1 (2). These are available from http://www.ercot.com.
Contrary to the expectations of many, it has been asserted that the quantity of ASs required by the ERCOT system operator has declined very slightly in recent years, despite a large increase in wind generation (Potomac Economics 2018, p. 37).1717 17 As noted by Potomac Economics, total AS requirements were 5300 MW in 2015, 4900 MW in 2016 and 4800 MW in 2018. This may, in large part, be due to improvements in ERCOT’s near-term forecasts of load and wind generation and a multitude of changes in the ERCOT Protocols (Andrade et al 2017). As suggested by Figure 6, there was a slight decrease in AS requirements between January 1, 2010 and December 31, 2017, as suggested by the negative slope coefficient in the trend line regression equation presented in this figure.
The amounts of ASs procured or awarded through the DAM may have declined or increased, depending on the period selected (Tsai 2018). Figure 7 suggests a slight increase in total ASs procured or awarded through the DAM in the period since the beginning of 2011. Changes in the procurement amounts may be explained by changes in requirements and changes in the self-arrangement of ASs, as noted in the following section.

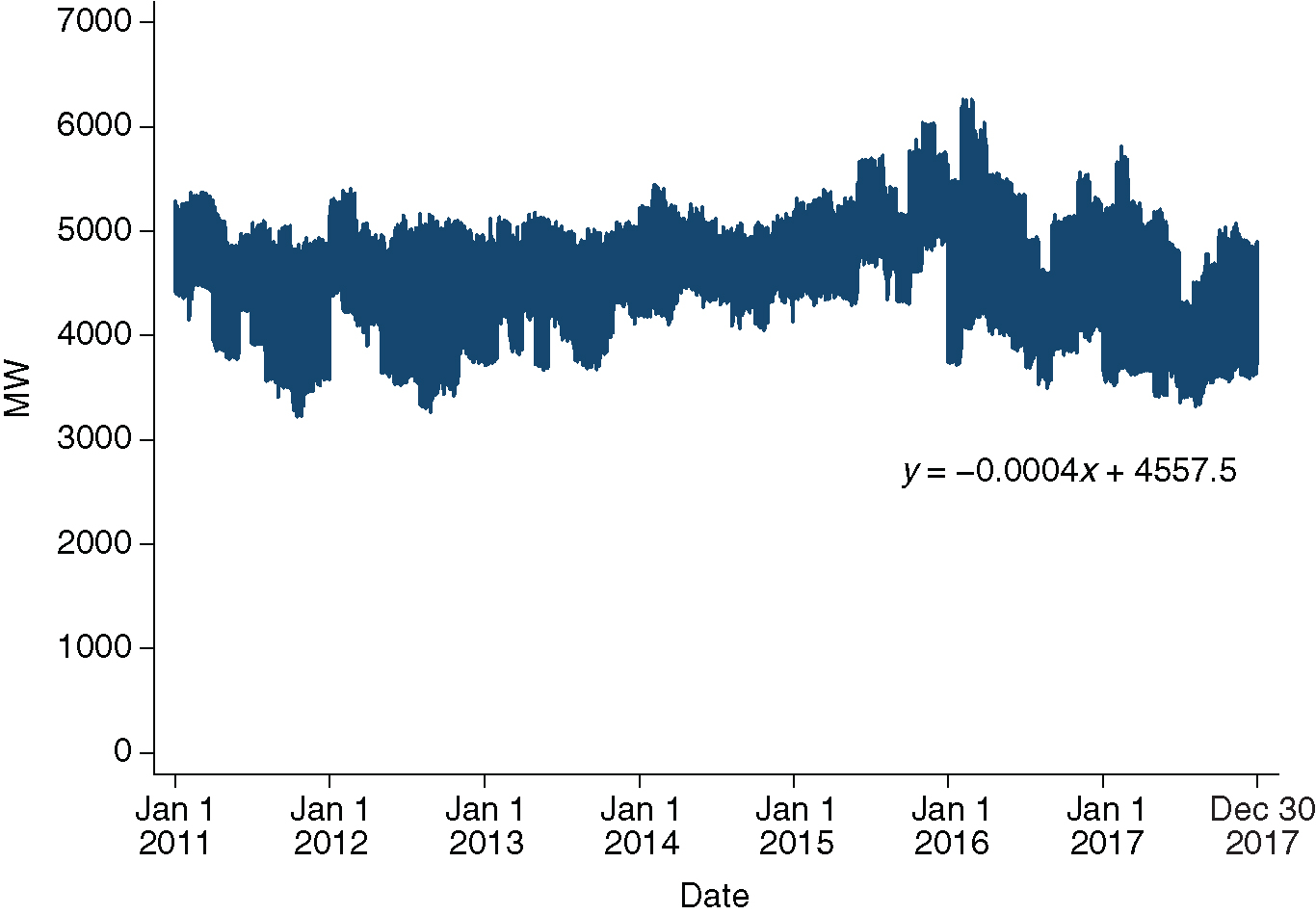
The market-clearing prices of ASs have generally declined. But how have the changes in AS market prices been affected by the changes in the quantities procured through the DAM, fluctuations in natural gas prices and other factors? This question is explored in the following sections.
2.3 Data
Obtained from the ERCOT website and through public data requests to ERCOT, our sample contains hourly data from January 1, 2011 to December 30, 2017 for the following variables, which are used in our price regressions described below.
- •
Generation (MWh) by fuel type for baseload nuclear power plants and wind farms for the ERCOT system.
- •
Hourly quantities required by ERCOT, offered to the DAM, and procured through the DAM of each AS (RRS, NSRS, REGUP and REGDN) in each hour (GW in each hour).1818 18 Some of our calculations may omit the small amounts that ERCOT procures through “supplemental auctions” for ASs. ERCOT has implemented supplemental ancillary services markets (SASMs, or supplemental auctions) 391 times since the start of the nodal market (see ERCOT 2018). ERCOT reports quantities in MW. The values in MW were converted to values in GW so that the coefficient estimates could be better illuminated.
- •
Hourly market-clearing price (USD per MW) of each AS as well as the price of energy (USD/MWh) procured (ie, awarded) through the DAM.
- •
For RRSs, the percentage or share of the required quantity provided by interruptible load resources.
- •
The system-wide forecast of load for each hour of the following day made available by ERCOT at the market at the start of each day’s DAM process.
- •
The system-wide forecast of wind generation for each hour of the following day made available by ERCOT to the market at the start of each day’s DAM procurement process.
In addition, the price of natural gas at the Henry Hub was obtained from the US Department of Energy (DOE) Energy Information Agency. Daily values were repeated for each hour of each day, and prices for days in which no trading took place (ie, weekdays and holidays) were based on prices for the prior trading day.
Our sample period closely matches the initiation of nodal pricing and the DAM on December 1, 2010. It begins on January 1, 2011 because coherent price data reflecting the new markets and zones was unavailable for December 2010. It ends on December 31, 2017, reflecting the data supplied to us by ERCOT at time of writing.
Figure 8 displays the pattern of AS prices over time. A simple trend analysis suggests a small decline in the prices of each AS over the period under consideration. Yet, simple trends are rather meaningless due to the large spikes indicated in this figure.


2.4 Price regressions
Our regression setup reflects that the DAMs for energy and ASs are interdependent because (a) many generation resources can offer energy or ASs, or both, to DAMs, and (b) resources are assigned to provide energy or ASs through ERCOT’s co-optimization routine. It also recognizes that DAM energy prices are mainly driven by such fundamental drivers as natural gas price, nuclear generation and day-ahead forecasts of system load and wind generation (Woo et al 2011; Zarnikau et al 2014a, 2016).
Let be the price for market (equal to 1 for DAM energy, 2 for RRSs, 3 for NSRSs, 4 for REGUP and 5 for REGDN) in hour () on day (). For the DAM energy price, the regression with random error is1919 19 We do not use the double-log specification because of the presence of zero and negative prices, whose natural logs are missing values that cause data gaps in our regression analysis. The values of all quantity variables representing generation and load were converted from MWh values to GWh values, so the coefficients associated with some of the variables could be better illuminated and all of these variables could be represented in the same units.
(2.1) |
In (2.1), coefficient is a time-varying intercept that captures the fixed effects of hour-of-day and month-of-year. Coefficient is the market-based heat rate that measures the DAM energy price increase due to a USD1/MMBtu increase in the natural gas price (Woo et al 2016b). Coefficient is the marginal price effect of baseload nuclear generation . Coefficient aims to capture the marginal price effect of ERCOT’s day-ahead load forecast . Coefficient measures the price-reduction (or merit-order) effect of the day-ahead forecast of wind generation . We do not consider other renewable generation types such as solar because of its small presence in Texas until recently and the absence of reliable data throughout the time frame of this analysis.
Equation (2.1) uses to capture the effects of ERCOT’s reliability-driven AS requirements on the DAM energy price, thus recognizing the ERCOT DAM’s energy–ASs interdependence ignored by prior price regression analyses for Texas (Woo et al 2011; Zarnikau et al 2014a, 2016). We expect to be positive because prices tend to increase as more ASs are required.
Let denote ERCOT’s AS procurement quantity of type () in hour on day ; let denote the portion of RRSs of provided by load resources; and let denote the total AS offer in hour on day . To allay concerns of estimation bias due to endogenous regressors, we reason that are predetermined variables that measure the AS procurement amounts based on ERCOT’s reliability requirements less LSEs’ total self-arranged amounts, known to ERCOT before its calculation of the DAM’s AS prices. We similarly reason that are predetermined variables, as they are made prior to ERCOT’s AS price determination.2020 20 This model does not use self-procured AS quantities as additional regressors because these quantities are endogenously determined by an LSE with self-arrangement capability based on its least-cost offer decisions. In particular, it will buy from the AS market when the AS price is below the per MW cost of self-arrangement. Thus, even if self-procured AS quantities have appeared in the model, DAM energy and AS prices move with the fundamental drivers and AS procurements according to the price regressions postulated here.
Reflecting the energy–AS markets’ interdependence and the predetermined nature of , and , our postulated AS price regressions with random errors are
(2.2) | ||||
(2.3) | ||||
(2.4) | ||||
(2.5) |
Coefficient in (2.2) is the intercept. Coefficient measures the DAM energy price’s effect on the RRS price.2121 21 Under the efficient market hypothesis (EMH), we expect to equal 1 based on the arbitrage behavior of suppliers. ERCOT’s reliability and operation constraints, however, imply that the EMH is unlikely to hold for all AS products. Hence, the change in a fundamental driver that alters the DAM energy price also impacts the RRS price. For example, is the RRS price effect of an increase in the natural gas price. Coefficient captures the demand-side price effect of . Finally, (2.2) uses coefficient to measure the supply-side effect of the hourly total RSS offer on the RRS price. As the interpretation of the remaining AS price regression is entirely analogous, it is omitted for brevity.
We tested a number of alternative models including dummy variables to represent relevant changes in market rules and modifications to the formulas used by ERCOT to calculate AS requirements; however, we concluded that the effects of these changes were already reflected in , leading to these dummy variables’ statistical insignificance.
Thanks to market interdependence, the random errors in (2.1)–(2.5) are contemporaneously correlated. As the hourly price data is likely serially correlated, we assume that the random errors follow an autoregressive () process, whose order is to be determined empirically. The endogenous DAM energy price appears as a regressor in (2.2)–(2.4). Thus, (2.1)–(2.5) form a system of simultaneous regressions, which can be readily estimated using the iterated three-stage-least square (IT3SLS) technique in the PROC MODEL of SAS software.
2.5 Data description
Descriptive statistics | Price correlation coefficients | ||||||||
---|---|---|---|---|---|---|---|---|---|
Variable: definition | Mean | SD | Minimum | Maximum | |||||
: DAM energy price ($/MWh) in hour on day |
31.817 | 55.090 | 1.188 | 2636 | 0.952 | 0.707 | 0.893 | 0.235 | |
: RRS price ($/MW/hr) in hour on day |
12.690 | 56.060 | 0.450 | 3000 | 0.953 | 0.790 | 0.931 | 0.284 | |
: NSRS price ($/MW/hr) in hour on day |
5.288 | 32.230 | 0.010 | 3000 | 0.707 | 0.790 | 0.741 | 0.190 | |
: REGUP price ($/MW/hr) in hour on day |
11.206 | 58.898 | 0.010 | 4999 | 0.893 | 0.931 | 0.741 | 0.278 | |
: REGDN price ($/MW/hr) in hour on day |
6.398 | 8.980 | 0 | 593 | 0.235 | 0.283 | 0.190 | 0.278 | |
: Henry Hub natural gas price ($/MMBtu) on day |
3.275 | 0.828 | 1.450 | 8.15 | 0.157 | 0.064 | 0.047 | 0.066 | 0.159 |
: nuclear generation (GWh) in hour on day |
4.491 | 0.795 | 0 | 7.590 | 0.030 | 0.022 | 0.023 | 0.020 | 0.069 |
: day-ahead system load forecast (GWh) in hour on day |
38.839 | 9.312 | 20.842 | 70.676 | 0.272 | 0.174 | 0.183 | 0.152 | 0.192 |
: day-ahead wind generation forecast (GWh) in hour on day |
4.736 | 2.882 | 0.143 | 28.612 | 0.140 | 0.053 | 0.074 | 0.058 | 0.169 |
: RRS procurement (GW/hr) in hour on day |
2.229 | 0.210 | 2.146 | 3.132 | 0.061 | 0.032 | 0.048 | 0.045 | 0.010 |
: share of RRS requirement met by load resources in hour on day |
0.482 | 0.037 | 0.211 | 0.512 | 0.048 | 0.059 | 0.063 | 0.051 | 0.008 |
: NSRS procurement (GW/hr) in hour on day |
1.630 | 0.328 | 0.496 | 2.804 | 0.014 | 0.022 | 0.067 | 0.021 | 0.064 |
: REGUP procurement (GW/hr) in hour on day |
0.440 | 0.146 | 0.176 | 1.139 | 0.078 | 0.038 | 0.034 | 0.075 | 0.110 |
: REGDN procurement (GW/hr) in hour on day |
0.396 | 0.118 | 0.156 | 0.859 | 0.018 | 0.009 | 0.006 | 0.011 | 0.262 |
: RRS requirement (GW/hr) in hour on day |
2.671 | 0.210 | 2.146 | 3.132 | 0.090 | 0.061 | 0.080 | 0.067 | 0.007 |
: NSRS requirement (GW/hr) in hour on day |
1.630 | 0.328 | 0.496 | 2.804 | 0.055 | 0.054 | 0.094 | 0.055 | 0.019 |
: REGUP requirement (GW/hr) in hour on day |
0.440 | 0.146 | 0.176 | 1.139 | 0.077 | 0.037 | 0.033 | 0.075 | 0.111 |
: REGDN requirement (GW/hr) in hour on day |
0.396 | 0.118 | 0.156 | 0.859 | 0.035 | 0.003 | 0.004 | 0.023 | 0.260 |
: RRS offer (GW/hr) in hour on day |
4.920 | 0.864 | 2.910 | 7.902 | 0.019 | 0.074 | 0.054 | 0.053 | 0.066 |
: NSRS offer (GW/hr) in hour on day |
4.209 | 1.011 | 2.343 | 27.287 | 0.016 | 0 | 0.003 | 0.009 | 0.019 |
: REGUP offer (GW/hr) in hour on day |
2.088 | 0.515 | 0.915 | 3.771 | 0.026 | 0.071 | 0.056 | 0.055 | 0.105 |
: REGDN offer (GW/hr) in hour on day |
1.722 | 0.612 | 0.697 | 3.704 | 0.004 | 0.038 | 0.024 | 0.021 | 0.052 |
Table 2 reports the descriptive statistics and price correlations of the variables used in our price regressions. All data series are found to be stationary at the 1% significance level based on the Phillip–Perron unit-root test (Phillips and Perron 1988), obviating concerns of spurious regressions due to nonstationary data that follows a random walk (Granger and Newbold 1974).
It is noteworthy that RRS prices tend to be greater than the prices of REGUP, since REGUP has stricter performance requirements. Some of the reasons explaining differences in the relative average prices of the two products include the following.2222 22 These explanations were provided through correspondence with Beth Garza of Potomac Economics.
- •
ERCOT requires much more RRS than REGUP (approximately 2800 MW for RRSs versus approximately 300 MW of REGUP).
- •
Generating units are limited in how much RRS they can provide. Historically, this limit was no more than 20% of a unit’s maximum capacity.
- •
While generation capacity providing RRSs is required to be frequency responsive, REGUP capacity is not required to have that capability.
The data series in Table 2 are volatile, with large standard deviations and wide ranges defined by the series’ minimum and maximum values. The DAM energy price is highly correlated with the RRS, NSRS and REGUP prices (), though not with the REGDN price (). The positive correlation coefficients () for the market prices of RRS, NSRS and REGUP suggest that these three market prices tend to move in tandem, thus affirming their interdependence. However, the positive correlations of the REGDN price with the DAM energy and other AS prices are weak (), presaging an empirical challenge in modeling REGDN price movements. Happily, the regressions results reported in Table 3 demonstrate that this challenge is an unwarranted concern.
DAM energy prices are positively correlated with natural gas prices and system loads but negatively correlated with wind generation output levels. Further, RRS, REGUP and REGDN prices are negatively correlated with AS offers. Still further, RRS prices are negatively correlated with the shares of RRS met by load resources.
Many of the remaining correlation coefficients in Table 2 fail to attain their expected signs, thus motivating our estimation of the price regressions given by (2.1)–(2.5), which controls for the effects of confounding variables and yields the generally reasonable empirics reported in the section below.
3 Results
Table 3 presents our IT3SLS regression results. The five price regressions have adjusted that range from 0.36 for REGDN to 0.93 for RRSs, indicating their eminently reasonable fit with the voluminous and noisy price data. The empirically determined AR order is because the parameter estimates for are all close to zero with dwindling statistical significance.2323 23 As an additional check, we assume errors to re-estimate the price regression system, yielding results that are virtually identical to those shown in Table 2. The estimated price regressions are empirically plausible because all coefficient estimates in Table 3 have the expected signs and are mostly (seventeen out of twenty-one) highly significant ().2424 24 Of the four coefficient estimates in the DAM energy price regression that are insignificant at the 1% level, three are significant at the 5% level. The estimate for nuclear generation, however, is highly insignificant ().
Equation (1): energy price ($/MWh) in hour on day . Equation (2): price ($/MW/hr) in hour on day . Equation (3): price ($/MW/hr) in hour on day . Equation (4): price ($/MW/hr) in hour on day . Equation (5): price ($/MW/hr) in hour on day .
Note: for brevity, this table omits the generally significant time-varying intercept estimates. The order is determined by their highly significant () parameter estimates. Increasing does not materially change the regression results because the resulting additional AR parameters are close to zero with dwindling statistical significance.]
Variable: definition | Eqn (1) | Eqn (2) | Eqn (3) | Eqn (4) | Eqn (5) |
---|---|---|---|---|---|
Adjusted | 0.745 | 0.930 | 0.623 | 0.808 | 0.360 |
Root mean square error (RMSE) |
27.92 | 14.90 | 19.86 | 25.94 | 7.04 |
: Henry Hub natural gas price ($/MMBtu) on day |
9.881 | ||||
: nuclear generation (GWh) in hour on day |
0.862 | ||||
: day-ahead load forecast (GWh) in hour on day |
2.657 | ||||
: day-ahead wind generation forecast (GWh) in hour on day |
1.671 | ||||
: RRS requirements (GW/hr) in hour on day |
3.618 | ||||
: NSRS requirements (GW/hr) in hour on day |
1.695 | ||||
: REGUP requirements (GW/hr) in hour on day |
20.754 | ||||
: REGDN requirements (GW/hr) in hour on day |
3.021 | ||||
: DAM energy price ($/MWh) in hour on day |
0.980 | 0.438 | 0.952 | 0.038 | |
: RRS procurement (GW/hr) in hour on day |
3.486 | ||||
: share of RRS requirement met by load resources in hour on day |
14.589 | ||||
: NSRS procurement (GW/hr) in hour on day |
5.632 | ||||
: REGUP procurement (GW/hr) in hour on day |
17.044 | ||||
: REGDN procurement (GW/hr) in hour on day |
31.343 | ||||
: RRS offer (GW/hr) in hour on day |
2.266 | ||||
: NSRS offer (GW/hr) in hour on day |
1.290 | ||||
: REGUP offer (GW/hr) in hour on day |
4.563 | ||||
: REGDN offer (GW/hr) in hour on day |
2.393 |
Focusing on the DAM energy price regression, we find that a USD1/MMBtu increase in natural gas price tends to increase the DAM energy price by about USD10.4/MWh, statistically close to a new combustion turbine’s engineering heat rate of about 9 MMBtu/MWh. This makes sense as Texas is a thermal system whose marginal fuel is natural gas with combustion turbines being the marginal resource at high demand levels.
The remaining coefficient estimates indicate that a 1 GW increase in system load would raise the DAM energy price by USD2.67/MWh on average, while the same 1 GW increase in wind generation would cause a DAM price decrease of $1.85/MWh on average. Nuclear generation’s estimated price effect is statistically insignificant (), which is half the size of wind generation’s. The difference in magnitude between the effects of wind and nuclear generation on prices may be due to differences in their temporal profiles. As expected, the DAM energy price increases with ERCOT’s AS requirements: a 1 GW increase’s estimated price effect is over USD20 per MW per hour for the REGUP requirement, and between USD1.69 per MW per hour and USD3.62 per MW per hour for the other three AS requirements.
Turning our attention to the AS price regressions, we find that a USD1/MWh increase in the DAM energy price tends to raise the RRS and REGUP prices by about a corresponding amount, nearly USD1 per MW per hour; this amount is much less for the NSRS and REGDN prices. The RRS price tends to decline with the share of RRS supplied by load resources.
The demand-side effects of a 1 GW increase in ERCOT’s AS procurements are USD3.49 per MW per hour for RRSs, $5.63 per MW per hour for NSRSs, USD17 per MW per hour for REGUP and USD31 per MW per hour for REGDN. The supply-side effects of a 1 GW increase in AS offers are AS price reductions of USD1.29 per MW per hour for NSRSs to USD4.56 per MW per hour for REGUP.
Eqn (1) | Eqn (2) | Eqn (3) | Eqn (4) | Eqn (5) | |
---|---|---|---|---|---|
Variable: definition | Period 1/Period 2 | Period 1/Period 2 | Period 1/Period 2 | Period 1/Period 2 | Period 1/Period 2 |
Adjusted | 0.755/0.716 | 0.931/0.946 | 0.569/0.848 | 0.928/0.431 | 0.419/0.346 |
Root mean square error (RMSE) |
41.69/15.45 | 22.86/6.39 | 30.59/8.38 | 23.13/28.70 | 6.67/7.47 |
: Henry Hub natural gas price ($/MMBtu) on day |
11.332/8.601 | ||||
: nuclear generation (GWh) in hour on day |
1.218/1.082 | ||||
: day-ahead load forecast (GWh) in hour on day |
4.548/1.941 | ||||
: day-ahead wind generation forecast (GWh) in hour on day |
2.119/1.040 | ||||
: RRS requirements (GW/hr) in hour on day |
12.048/13.642 | ||||
: NSRS requirements (GW/hr) in hour on day |
0.963/0.150 | ||||
: REGUP requirements (GW/hr) in hour on day |
16.9333/20.161 | ||||
: REGDN requirements (GW/hr) in hour on day |
4.715/1.931 | ||||
: DAM energy price ($/MWh) in hour on day |
0.992/0.961 | 0.411/0.626 | 0.977/0.823 | 0.049/0.015 | |
: RRS procurement (GW/hr) in hour on day |
2.015/12.03 | ||||
: share of RRS requirement met by load resources in hour on day |
6.161/36.017 | ||||
: NSRS procurement (GW/hr) in hour on day |
5.939/2.670 | ||||
: REGUP procurement (GW/hr) in hour on day |
9.500/25.036 | ||||
: REGDN procurement (GW/hr) in hour on day |
21.147/42.507 | ||||
: RRS offer (GW/hr) in hour on day |
0.224/0.736 | ||||
: NSRS offer (GW/hr) in hour on day |
0.890/0.620 | ||||
: REGUP offer (GW/hr) in hour on day |
0.050/8.665 | ||||
: REGDN offer (GW/hr) in hour on day |
1.422/4.974 |
As correctly noted by a reviewer, one might expect many of the relationships among these variables to evolve over this long seven-year period, as the market has evolved. Since we could not identify any obvious point of structural change in this market during the time period analyzed, we simply divide the estimation in this period in half. Thus, period 1 encompasses January 1, 2011 to June 30, 2013 and period 2 covers July 1, 2013 to December 30, 2017. Indeed, some of the relationships significantly changed between these two time periods, as noted in Table 4. The impact of the day-ahead load forecast on DAM energy prices has declined. The impact of the DAM energy price on the price of REGDN has gone from positive to negative. The price reductions effect on RRSs from a 1% increase in the share of RRS provided by interruptible loads increased by nearly sixfold from the first period to the second. Chow tests for subsample stability confirm the presence of some structural changes between these two periods. Yet, it is noteworthy that the effect of the wind generation forecast on DAM prices did not change significantly at the 5% level of statistical significance; nor were the changes in the coefficients of the gas price and nuclear generation variables significant.
4 Discussion
The empirics reported in Section 3 have important implications for generation investments and electricity bills. Specifically, the DAM price regression’s coefficient estimate for wind generation affirms the order merit effect that weakens the investment incentive for natural-gas-fired generation but helps reduce electricity bills (Liu et al 2016; Woo et al 2012, 2016b). If rising wind generation also increases ERCOT’s AS requirements, its order merit effect on the DAM energy price diminishes.
The AS regressions’ coefficient estimates suggest that rising wind generation tends to reduce AS prices because of its merit order effect on the DAM energy price, which in turn causes the AS prices to decline. To be sure, if rising wind generation also increases in ERCOT’s AS procurement quantity, it shrinks these AS price declines. A substantive question thus arises: have ERCOT’s AS procurements been rising in light of the rapid wind generation development in Texas?
The answer appears to be no based on Figure 7, which indicates that procurement quantities have remained quite stable despite the wind generation capacity expansion in the last decade. This stability may be due partly to ERCOT’s improved accuracy in forecasting wind generation and total system generation, and partly to a variety of protocol changes (Andrade et al 2017). Declining natural gas prices that cut the DAM energy prices also contribute to ERCOT’s stable AS prices.
Notwithstanding the above findings, we would be remiss had we failed to acknowledge the potential risk that ERCOT’s AS cost could escalate in the future. This risk can be attributed to the very high marginal procurement costs of REGUP and REGDN that are used to counter the rapid fluctuations in intermittent generation resources such as wind. Further, 4 GW of coal capacity was retired in early 2018, which may also result in decreases in AS offer quantities and hence amplify the potential risk of AS cost escalation.2525 25 For instance, the average DAM market-clearing price of REGUP increased to USD320/MW per hour in July 2018. The average REGDN price also increased to USD290/MW per hour. This price spike in AS may reflect the tight AS supply condition. Interestingly, the DAM average energy price stayed below USD35/MWh, except during the few hours in which the price skyrocketed and exceeded USD1000/MWh due to extreme high demand and low wind production.
5 Conclusions
Based on an econometric analysis of AS prices set in ERCOT’s DAM from January 1, 2011 to December 30, 2017, we find the following.
- •
ERCOT’s requirements for ASs have proven remarkably stable over the seven years examined here, despite a large expansion in wind generation capacity. In the 2011–17 period, a very slight negative trend in requirements may be seen, contrary to early predictions that increased dependence on intermittent renewable energy generation would prompt increases in ERCOT’s AS requirements.
- •
The pattern of quantities of ASs procured via ERCOT’s formal market for ASs mirrors the pattern exhibited by requirements, though the values are smaller due to the ability of some LSEs to self-arrange their obligations. Thus, the quantities procured through the DAM have also proven quite stable.
- •
The marginal cost of acquiring additional REGUP and REGDN capacity through ERCOT’s DAM is quite high on average (despite the low average cost of these services), after controlling for the effects of other variables. This suggests that any increase in the requirements for these services may come at a high cost. The need for these services is sensitive to the levels of wind generation and forecasting error.
- •
We also find that the markets for RRSs and REGUP are efficient, in the sense that a one-unit increase in DAM energy prices tends to increase RRS and REGUP prices by roughly one unit. This is to be expected in an efficient market where resources are co-optimized, since generating units that set the market-clearing prices of RRSs and REGUP could alternatively be used to provide energy.
- •
An increase in DAM energy prices will lead to a lesser increase in NSRS prices, reflecting that providers of this service are not necessarily online and burning fuel, and thus incur lower costs to provide this service.
- •
Increases in the share of RRSs provided by load resources lead to lower prices for RRS because interruptible loads offer their demand reduction capacity at very low prices in the RRS market. Hence, an increase in load participation can reduce RRS market prices, which are typically set by generating units, in a manner similar to how increased baseload generation can reduce energy prices via a merit order effect.
In summary, our documentation of the market price behavior of ERCOT’s DAM energy and AS prices suggests that wind energy development has reduced these prices in the last seven years, without materially raising ERCOT’s AS requirement and procurement. Additional data collection and analysis in the coming years will tell us whether this suggestion’s empirical validity continues to hold in the future.
Declaration of interest
The authors report no conflicts of interest. The authors alone are responsible for the content and writing of the paper.
References
- Andrade, J., Baldick, R., and Dong, Y. (2017). Analysis of ERCOT regulation-up and regulation-down operational reserves. In Proceedings of the IEEE Texas Power and Engineering Conference, College Station, TX, February 9–10. IEEE (https://doi.org/10.1109/TPEC.2017.7868266).
- Argonne National Laboratory (2016). Survey of US ancillary services markets. Report ANL/ESD-16/1. URL: https://bit.ly/2BCUhKb.
- Distributed Energy Financial Group (2015). The annual baseline assessment of choice in Canada and the United States (ABACCUS). Report. URL: https://bit.ly/1VLNyAQ.
- ERCOT (2013). Future ancillary services in ERCOT. Concept Paper.
- ERCOT (2018). Study of the operational improvements and other benefits associated with the implementation of real-time co-optimization of energy and ancillary services. Report, June 29.
- Exeter Associates and GE Energy (2012). Review of industry practice and experience in the integration of wind and solar generation. Task Report, November. URL: https://bit.ly/21b6oE5.
- GE Energy (2008). Analysis of wind generation impact on ERCOT ancillary services requirements. Report, March 28, ERCOT. URL: https://bit.ly/2tmCIJZ.
- Granger, C. W. J., and Newbold, P. (1974). Spurious regressions in econometrics. Journal of Econometrics 2, 111–120 (https://doi.org/10.1016/0304-4076(74)90034-7).
- Heffner, G., Goldman, C., and Kirby, B. (2007). Loads providing ancillary services: review of international experience. Report 11231, May, Ernest Orlando Lawrence Berkeley National Laboratory. URL: https://bit.ly/2TbgSo2.
- Liu, Y., Woo, C. K., and Zarnikau, J. (2016). Ex post payoffs of a tolling agreement for natural-gas-fired generation in Texas. The Journal of Energy Markets 9(1), 21–45 (https://doi.org/10.21314/JEM.2016.139).
- National Renewable Energy Laboratory (2011a). Operating reserves and variable generation. Technical Report NREL/TP-5500-51978, NREL. URL: https://bit.ly/2CCPsQN.
- National Renewable Energy Laboratory (2011b). Effective ancillary services market designs on high wind power penetration systems. Conference Paper NREL/CP-5500-53514, NREL. URL: https://bit.ly/2TeSD8x.
- Oren, S. S. (2001). Design of ancillary service markets. In Proceedings of the 34th Annual Hawaii International Conference on System Sciences. IEEE (https://doi.org/10.1109/HICSS.2001.926283).
- Phillips, P. C. B., and Perron, P. (1988). Testing for a unit root in time series regression. Biometrika 75, 335–346 (https://doi.org/10.1093/biomet/75.2.335).
- Potomac Economics (2018). 2017 state of the market report for the ERCOT electricity market. Report, May. URL: https://bit.ly/2W5V6nk.
- Sioshansi, F. P. (2013). Evolution of Global Electricity Markets: New Paradigms, New Challenges, New Approaches. Elsevier.
- Stoft, S. (2002). Power System Economics. Wiley–IEEE Press (https://doi.org/10.1109/9780470545584).
- Tsai, C. H. (2018). When ancillary services decrease with more wind in ERCOT. Electricity Journal 31(5), 38–45 (https://doi.org/10.1016/j.tej.2018.06.002).
- Woo, C. K., Horowitz, I., Moore, J., and Pacheco, A. (2011). The impact of wind generation on the electricity spot-market price level and variance: the Texas experience. Energy Policy 39, 3939–3944 (https://doi.org/10.1016/j.enpol.2011.03.084).
- Woo, C. K., Horowitz, I., Horii, B., Orans, R., and Zarnikau, J. (2012). Blowing in the wind: vanishing payoffs of a tolling agreement for natural-gas-fired generation of electricity in Texas. Energy Journal 33(1), 207–229 (https://doi.org/10.5547/ISSN0195-6574-EJ-Vol33-No1-8).
- Woo, C. K., Moore, J., Schneiderman, B., Olson, A., Jones, R., Ho, T., Toyama, N., Wang, J., and Zarnikau, J. (2015). Merit-order effects of day-ahead wind generation forecast in the hydro-rich Pacific Northwest. Electricity Journal 28(9), 52–62 (https://doi.org/10.1016/j.tej.2015.10.001).
- Woo, C. K., Moore, J., Schneiderman, B., Ho, T., Olson, A., Alagappan, L., Chawla, K., Toyama, N., and Zarnikau, J. (2016a). Merit-order effects of renewable energy and price divergence in California’s day-ahead and real-time electricity markets. Energy Policy 92, 299–312 (https://doi.org/10.1016/j.enpol.2016.02.023).
- Woo, C. K., Horowitz, I., Zarnikau, J., Moore, J., Schneiderman, B., Ho, T., and Leung, E. (2016b). What moves the ex post variable profit of natural-gas-fired generation in California? Energy Journal 37(3), 29–57.
- Woo, C. K., Chen, Y., Olson, A., Moore, J., Schlag, N., Ong, A., and Ho, T. (2017). Electricity price behavior and carbon trading: new evidence from California. Applied Energy 204, 531–543.
- Woo, C. K., Chen, Y., Zarnikau, J., Olson, A., Moore, J., and Ho, T. (2018). Carbon trading’s impact on California’s real-time electricity market prices. Energy 159, 579–587 (https://doi.org/10.1016/j.energy.2018.06.188).
- Zarnikau, J. (2005). A review of efforts to restructure Texas’ electricity market. Energy Policy 33, 15–25 (https://doi.org/10.1016/S0301-4215(03)00193-9).
- Zarnikau, J., Woo, C. K., and Baldick, R. (2014a). Did the introduction of a nodal market structure impact wholesale electricity prices in the Texas (ERCOT) market? Journal of Regulatory Economics 45, 194–208 (https://doi.org/10.1007/s11149-013-9240-9).
- Zarnikau, J., Woo, C. K., Gillett, C., Ho, T., Zhu, S., and Leung, E. (2014b). Day-ahead forward premiums in the Texas electricity market. The Journal of Energy Markets 8(2), 1–20 (https://doi.org/10.21314/JEM.2015.126).
- Zarnikau, J., Woo, C. K., and Zhu, S. S. (2016). Zonal merit-order effects of wind generation development on day-ahead and real-time electricity market prices in Texas. The Journal of Energy Markets 9(4), 17–47 (https://doi.org/10.21314/JEM.2016.153).
Only users who have a paid subscription or are part of a corporate subscription are able to print or copy content.
To access these options, along with all other subscription benefits, please contact info@risk.net or view our subscription options here: http://subscriptions.risk.net/subscribe
You are currently unable to print this content. Please contact info@risk.net to find out more.
You are currently unable to copy this content. Please contact info@risk.net to find out more.
Copyright Infopro Digital Limited. All rights reserved.
As outlined in our terms and conditions, https://www.infopro-digital.com/terms-and-conditions/subscriptions/ (point 2.4), printing is limited to a single copy.
If you would like to purchase additional rights please email info@risk.net
Copyright Infopro Digital Limited. All rights reserved.
You may share this content using our article tools. As outlined in our terms and conditions, https://www.infopro-digital.com/terms-and-conditions/subscriptions/ (clause 2.4), an Authorised User may only make one copy of the materials for their own personal use. You must also comply with the restrictions in clause 2.5.
If you would like to purchase additional rights please email info@risk.net