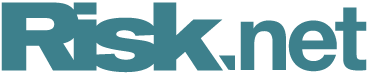
Exploiting causal biases in market impact models
Model calibration gains efficiency by including biased but adjusted trading data
The efficiency of the execution of a large trade depends on minimising its market impact. Traders need to find the optimal balance between speed of execution and the price move that execution causes.
The problem is as old as trading itself and common to all big market players. The literature on the subject over recent decades is vast, but no holy grail has been found.
Typically, to calibrate a market impact model, firms only have data of their own trading. The observed returns are partly attributable to their trading and partly attributable to the price move that would have happened anyway. Through A/B tests, in which inputs are randomised, firms can assess the effect of a trade’s impact with and without the presence of independent market moves, but the resulting calibration may be suboptimal.
If models are calibrated without managing the independent price moves or filtering them out, trades will appear more expensive. If a buy trade is executed while the market is going up independently of the trade, impact models can be tricked into considering the whole move as having been the impact of the trade.
The paper deals primarily with the estimation of the market impact in the presence of alpha
NIcholas Westray, AllianceBernstein
“As a result, when you subsequently go to decide how much you want to trade, you’re going to size your trade smaller than it should be,” says Nicholas Westray, head of execution research in AllianceBernstein’s hedge fund and multi-asset solutions group and visiting researcher in financial machine learning at New York University’s Courant Institute of Mathematical Sciences. Ultimately, that will affect the strategy’s P&L.
Isolating the trade’s impact from the independent market move is one of the challenges when it comes to calibrating impact models. One way to overcome the issue is to execute a few additional trades that do not exhibit price moves. That can be done, for example, by submitting random trades, which on average will have no independent moves. These enable the trader to assess the impact of the trade in question and calibrate the model accordingly. However, this approach is costly because each additional trade comes with its own transaction fees.
Westray believes the cost can be reduced significantly. In a paper written with Kevin Webster – an adjunct assistant professor at Columbia University, visiting reader at Imperial College London and a former colleague during Westray’s time at Deutsche Bank – he has developed a method to calibrate the impact model with fewer datapoints.
“The paper deals primarily with the estimation of the market impact in the presence of alpha,” he says, referring to the price moves that occur independently of a firm’s own trading activity.
Cause and defect
Westray and Webster identified the crux of the issue as a bias created by the causal relationships linking alpha and the size and returns of trades. Alpha drives the size of the trades, and size and alpha together drive the returns. It is this chain of causal relationships that causes the bias.
They argue that the bias can be handled by using causal regularisation, a technique introduced in 2019 by Dominik Janzing that is designed to reduce the number of coefficients in a regression model and facilitate the identification of causal links. Janzing argued that by applying regularisation it is possible to get better causal models, or models with less causal bias.
Westray says that with his and Webster’s methodology “this means we fit our model on all of the training data, and then use the control data to calibrate the regularisation hyperparameter, leading to an improved output”.
He adds that regularisation parameters are more stable than coefficients because there is more stationarity in hyperparameters – the parameters that define the model’s structure – than there is in regular parameters, and that less control data is therefore required.
As Westray and Webster illustrate in their paper, if the impact model is fitted to the trading data, the large number of datapoints leads to a low standard deviation but the estimate is affected by bias. Fitting only to unbiased data removes that bias, but the low number of datapoints means the estimation error is higher. Fitting to a combined set of biased and unbiased data – in which the biased is adjusted with causal regularisation – allows for a fitting with low standard deviation. “We show that if you estimate on the biased data, and then use the control data to correct the bias, you can get best-in-class outcomes,” says Westray.
To give an example of how the methodology might be applied, a research institution that developed 20 new strategies might want to assess them with A/B testing – an approach that could take a long time and for which the outcomes might be biased. “Using this methodology means you need less control data to establish the effect that you’re looking for,” says Westray. “We view it as a tool for doing more efficient A/B tests.”
When he was approached by Westray, Webster, like many in the buy-side industry, had been interested for some time in finding a way to deal with alpha when estimating a transaction cost model. “In 2022, Kevin came to me and said he had a new approach for looking at this,” he says. “And he just needed some simulated data to prove its efficacy. I had been working on a simulator, so I was easily able to generate the data for him, and that’s where the collaboration came from.”
These are early days and the methodology has yet to be tested in a live trading environment. Nevertheless, Westray feels confident about the approach, given the results it has delivered thus far: “I’m optimistic about the potential in practice.”
Editing by Daniel Blackburn
Only users who have a paid subscription or are part of a corporate subscription are able to print or copy content.
To access these options, along with all other subscription benefits, please contact info@risk.net or view our subscription options here: http://subscriptions.risk.net/subscribe
You are currently unable to print this content. Please contact info@risk.net to find out more.
You are currently unable to copy this content. Please contact info@risk.net to find out more.
Copyright Infopro Digital Limited. All rights reserved.
As outlined in our terms and conditions, https://www.infopro-digital.com/terms-and-conditions/subscriptions/ (point 2.4), printing is limited to a single copy.
If you would like to purchase additional rights please email info@risk.net
Copyright Infopro Digital Limited. All rights reserved.
You may share this content using our article tools. As outlined in our terms and conditions, https://www.infopro-digital.com/terms-and-conditions/subscriptions/ (clause 2.4), an Authorised User may only make one copy of the materials for their own personal use. You must also comply with the restrictions in clause 2.5.
If you would like to purchase additional rights please email info@risk.net
More on Views
Degree of influence 2024: volatility and credit risk keep quants alert
Quantum-based models and machine learning also contributed to Cutting Edge’s output
Podcast: Alexandre Antonov turns down the noise in Markowitz
Adia quant explains how to apply hierarchical risk parity to a minimum-variance portfolio
UBS’s Iabichino holds a mirror to bank funding risks
Framing funding management as an optimal control problem affords an alternative to proxy hedging
Podcast: Alexei Kondratyev on quantum computing
Imperial College London professor updates expectations for future tech
Quants mine gold for new market-making model
Novel approach to modelling cointegrated assets could be applied to FX and potentially even corporate bond pricing
Quants dive into FX fixing windows debate
Longer fixing windows may benefit clients, but predicting how dealers will respond is tough
Podcast: Piterbarg and Nowaczyk on running better backtests
Quants discuss new way to extract independent samples from correlated datasets
BofA quants propose new model for when to hold, when to sell
Closed-form formula helps market-makers optimise exit strategies