
This article was paid for by a contributing third party.More Information.

Making machine learning work for AML
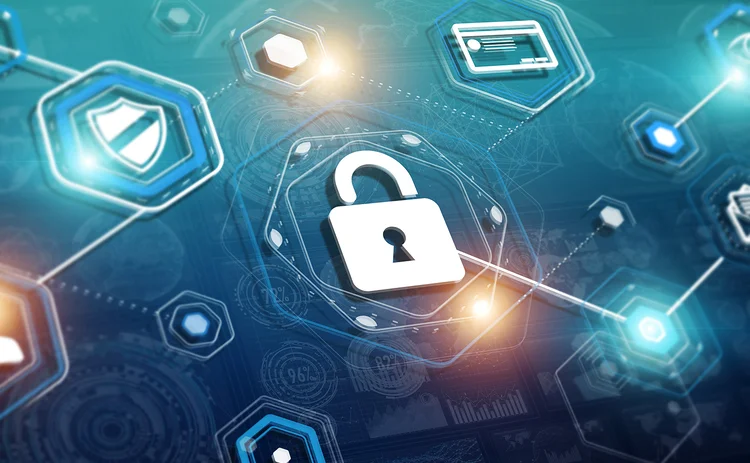
Banks’ anti-money laundering teams are starting to utilise machine learning to combat financial criminals. Risk hosted a webinar in association with NICE Actimize to explore whether these bots can be trusted
The panel
- Ted Sausen, Director and AML subject matter expert, NICE Actimize
- Evan Weitz, Managing director and regional head of controls, Standard Chartered Bank
- Jayati Chaudhury, Global investment banking lead for AML transaction monitoring, Barclays
- Moderator: Duncan Wood, Editor-in-chief, Risk.net
The long war between banking and money laundering has led banks to deploy a new generation of smart weapons. Financial institutions are rolling out machine learning innovations in particular to beat financial crime through greater accuracy and efficiency.
Banks are responding not just to criminals but to regulators, which now enforce tougher penalties and charge greater fines for breaches. Fearing supervisors’ punishments and reputational damage, management has become more zealous in its anti-money laundering (AML) efforts than ever before.
US regulators have taken the lead, while European supervisors play catch-up. AML fines in Europe and the UK totalled $214 million from 2014 to 2017, with those in the US at $1.96 billion, according to data from ORX. Fast forward to last year and, during the first three quarters of 2018, fines in the UK and Europe reached $918 million, compared with just over $1 billion in US penalties.
Magic bullet?
Amid this pressurised compliance environment, machine learning is being touted as a ‘magic bullet’ for AML teams. In a snap survey of several hundred webinar listeners, one-third said they thought machine learning would be “widely used and transformative”, but nearly half worried its huge potential was let down by a lack of transparency.
“I don’t think it’s a magic bullet, but do I think its use is long overdue,” said Ted Sausen, director and AML subject matter expert at NICE Actimize. “Machine learning isn’t new as a concept, but it is new in the AML space.”
He added: “What’s clear is that there’s a place for it to complement our traditional approaches. We have a lot of false positives and, if we look at the amount of money laundering that’s still happening and how much we’re capturing, something has to be done.”
Jayati Chaudhury, global investment banking lead for AML transaction monitoring at Barclays, agreed that machine learning should be used in conjunction with existing AML efforts.

“It’s a big step forward and it’s the right way to go, but I don’t believe it has the entire solution all by itself, simply because the solutions are not yet mature enough,” she said. “It should be something to augment our current processes and it needs to be well understood.”
Chaudhury also noted the wide range of areas to be covered within AML – most obviously transaction monitoring, including investigation of alerts, and also know-your-customer (KYC), which involves customer due diligence such as checks for source of funds.
The enduring importance of the role human judgement plays in these processes was emphasised by Evan Weitz, managing director and head of controls in Europe and the US at Standard Chartered.
“I think the technology has tremendous potential, but it can never entirely replace human judgement,” Weitz said, agreeing with Chaudhury about the need to augment existing defences with artificial intelligence (AI) and particularly to use technology to direct resources.
“At the heart of any AML programme is the simple exercise of good human judgement as to what appears suspicious, what doesn’t, what is within the risk profile, and what isn’t,” he continued. “I think it is something that can make us much more effective at our jobs.”
Regulatory zeal
Even the watchdogs are talking up innovation, but details are lacking about how they view the role of AI or how they might respond to a bank that gets it wrong.
On December 3, 2018 four US prudential regulators, together with the Financial Crimes Enforcement Network (Fincen), came together to encourage banks to “consider, evaluate and, where appropriate, implement innovative approaches to meet their AML compliance obligations”. Banks are considering how to use machine learning in their interpretation of that statement.
“There is encouragement and there is no penalty for trying new solutions, but there is also the risk of limited understanding and the factor of a lack of transparency in using machine learning solutions. It may be a challenge to explain to regulators in the absence of this transparency,” Chaudhury said.
Weitz weighed in with his experience as a former US federal prosecutor, suggesting that authorities may lack awareness of the weight of resources deployed at detection scenarios, which machine learning would also be deployed at.
“That said, the US regulators have got much better over the past few years at getting down in the weeds and working with the banks to make the programme better,” said Weitz.
“However, we have to be realistic that there’s always going to be healthy scepticism by regulators whenever an institution comes to them with something with the ultimate aim of being more efficient and saving the bank money,” he added.
One thing about the regulators’ statement that is not ambiguous is the desire for innovation. The words ‘innovative’ and ‘innovation’ appear multiple times within the joint statement.

Weitz lauded the tone of the document. “I thought the joint statement was absolutely fantastic,” he said. “If you haven’t seen it already, I’d encourage you to go to the Fincen website. To me, this was a bit of a game changer, because previously there had never been this formally recognised desire to innovate.”
Weitz noted one paragraph in particular, in which the regulators encouraged banks to try using AI for monitoring transactions, but not to worry about supervisors assuming their previous AML processes were deficient if the new technology comes up with different answers, but rather to welcome the progress. “We’re being encouraged to fix things, and to get better. I can’t overstate the importance of this,” he added.
Sausen agreed there has been a widespread fear to uncover something that would require a huge backward-looking compliance exercise. Secondly, he noted this could be advantageous to regulators, as they have millions of suspicious activity reports (SARs) piling up on their own desks.
Reducing false positives
Estimates of the percentage of money laundering that is actually uncovered make for depressing reading – somewhere between 1% and 5%. The sheer volume of ‘dirty money’ escaping the net – combined with the rate of false positives in AML efforts – means maintaining the status quo cannot be an option.
Weitz focused on the inefficiency of having so many false positives, pointing to his own firm’s challenges in transaction monitoring. Nine in every 10 alerts are dealt with in the first stages of monitoring, he explained, first by an offshore team. The small remaining percentage is then escalated to his team, which is able to satisfy about two-thirds of those remaining alerts, leaving a small number that wind up as SARs.
“Between 1% and 2% of my AML alerts are becoming SARs or something that is actionable,” he explained. “I question why as, an industry, we’re satisfied with that, if 98% of our time is spent on cases that ultimately turn out not to be suspicious,” Weitz said.
He said Standard Chartered had explored using machine learning within a risk-scoring context of red flags and parameters for suspicion. The team can then focus on the remaining cases.
“Machine learning and AI can be most transformative by helping figure out where we should be looking and to help identify and hibernate the 98% of cases that are false positives. That would allow us to put more resources into the 2% of cases that are more likely to be suspicious,” said Weitz.
“It allows us to focus more resources into some of our manual programmes, or we’re looking at things like subpoenas, working with law enforcement and launching more proactive reviews, which can be far more risk-relevant than detection scenarios to our SAR disposition ratio,” he added.
Test pilots
Machine learning for AML has to begin somewhere, but benchmarking it can be problematic in the early years. A poll of webinar listeners suggested, when piloting new applications, that reducing false-positive alerts was the most popular place to start, with anomaly detection and segmentation chosen by others.
Sausen agreed with putting an onus on segmentation, as well as the headliner of cutting false positives. Segmentation has been relatively neglected so far, he suggested.
“Tuning can be painful, hard work and can involve a lot of ‘guesstimation’ to tweak thresholds here and there, but model tuning and segmentation are two key areas at which you should be looking,” he said.
“That is one way to fix your programme: get your segmentation and tuning right and then start looking at your machine learning to find other anomalies that a traditional scorecard or rules-based model can’t detect,” Sausen continued. “We’re using machine learning to tune segmentation and tune the model to identify the rules and thresholds that need to be changed,” he added.
In an environment where false positives have crept up past 95%, Sausen emphasised the importance of above- and below-the-line testing to reduce the false-positive rate and for anomaly detection. If segmentation is undertaken incorrectly, peer groups are also wrong, directly impacting the model’s ability to appropriately identify risk.
At Barclays, Chaudhury said she has started exploring solutions that help detect changes in behaviour. Pilots are too early for like-for-like result comparisons, she explained – particularly for transaction monitoring – adding that new technology is
only augmenting rather than replacing rules- based monitoring.
She was unwilling to judge success in reducing false-positive rates at this point, but emphasised that fresh linkages present a bigger picture. “What we see is the benefit of being able to view a network that was not visible to us before,” she said.

“It’s not ready to be benchmarked yet, but I think it will be helpful to benchmark the capability itself as to whether you are able to detect those connections that bring suspicious activity to the forefront to be investigated,” said Chaudhury.
“What we’ve been missing out in monitoring are the new patterns that can emerge, because the perpetrators of the crime are usually one step ahead of us. Any new patterns that emerge may or may not have an apparent connection, but when the data mining layer is in there and the machine learning solutions are working behind the scenes to detect those connections, suspicious actors will surface,” Chaudhury added.
Benchmarking
With machine learning’s AML roll-out still at an early stage, benchmarking is tough without help between institutions, Weitz suggested. He held informal discussions with colleagues at other banks in search of solid benchmarks.
“We really were out in the woods and alone on this,” he said. “We had to do a lot of internal testing to validate that this was worth doing, as opposed to more established technologies, with established benchmarks to compare yourself against.”
Transaction screening is the focus of a machine learning programme at Standard Chartered in Singapore. The aim is to reduce false positives, Weitz explained, by relying on a mix of human interpretation and machine learning to succeed.
“To be clear, when I say ‘screening’ I mean whether we’re going to stop live payments and reject it for sanctions or other reasons, rather than transaction monitoring review after transactions have been processed,” said Weitz.
“The machine will essentially learn after we’ve approved a certain number of false positives that are no longer treated necessarily as a false positive. Like so many other transaction-based banking products, it is very much focused on how much time it takes to process transactions, which can have tremendous advantages for our business,” he added.
Sausen suggested the different ways nascent machine learning applications are not the same as models. The different ways machine learning pilot applications are being applied in their early stages means they should be treated differently to other models, such as those for credit risk, “We’re using it for guidance at this point,” he said. “It’s complementing our solution and helping us prioritise. It gets referred to as a black box, because it’s much harder to test than a scorecard type of scenario.”
Agreeing that applications are not the same as models, Chaudhury suggested there is a mistaken tendency among model validators to test them on standard dimensions, such as benchmarking productivity. “It is hard to benchmark a transaction monitoring or surveillance kind of model. There is no industry benchmark,” she added.
Explain yourself
There will be increased pressures around transparency and to provide answers to internal and external stakeholders once machine learning applications or solutions go live beyond isolated trials and test pilots.
“The transparency and explainability of it is going to be crucial for any model owners like ourselves to be able to rationalise to whoever is asking the questions, be it an auditor, model validator or a regulator,” Chaudhury stressed.
Weitz suggested AML would need to sell the benefits of using machine learning for AML. “The first questions asked by audit or any regulator are: ‘What are you missing and how are you accounting for that?’” he said.
There are likely to be instances where a machine learning approach fails to detect all of the suspicious behaviour picked up by previous processes for detection scenarios, he admitted, allowing a would-be SAR to escape the net.
“You have to have a stated risk tolerance for what you as an institution are going to accept. For Standard Chartered, it’s proprietary information, but I can tell you it’s in the single digits,” he said.
“You have to bring regulators and audit on board that you’re not always going to have 100% coverage, but you’re making a risk-based decision, in exchange for efficiency, to redeploy resources to other more risk-relevant areas, and that you’re willing to accept at least a couple of misses. This is an easier sell for transaction monitoring than for screening,” added Weitz.
Chaudhury noted that explanations to regulators may differ between institutions, with factors including the business landscape, as well as firms’ client and product mixes.
“That explainability starts with how you have determined your risk, how you’ve determined the typologies that apply to you, and therefore the type of scenarios you have to come up with,” she said.
Black box type solutions create more challenges since banks generally do not develop the code, and thereby create dependency on vendors as well as model owners, she noted. Strong relationships with vendors will be crucial, Chaudhury said.
“Transparency will come as there is more understanding towards what firms like us are trying to achieve with machine learning and AI rather than viewing them as solutions to simply replace what we have,” Chaudhury said. “I think a constant dialogue should be encouraged by the vendors.”
Sausen emphasised that NICE Actimize was ready to focus on problems before suggesting solutions: “What we need to do on the vendor side is look at what the financial institution’s problem is, find the problem, and then look at particular ways to solve it, rather than saying machine learning always has to be the answer.”
Watch the full webinar, Using machine learning for AML
The panellists were speaking in a personal capacity. The views expressed by the panel do not necessarily reflect or represent the views of their respective institutions.
Sponsored content
Copyright Infopro Digital Limited. All rights reserved.
As outlined in our terms and conditions, https://www.infopro-digital.com/terms-and-conditions/subscriptions/ (point 2.4), printing is limited to a single copy.
If you would like to purchase additional rights please email info@risk.net
Copyright Infopro Digital Limited. All rights reserved.
You may share this content using our article tools. As outlined in our terms and conditions, https://www.infopro-digital.com/terms-and-conditions/subscriptions/ (clause 2.4), an Authorised User may only make one copy of the materials for their own personal use. You must also comply with the restrictions in clause 2.5.
If you would like to purchase additional rights please email info@risk.net