
The wild world of credit models
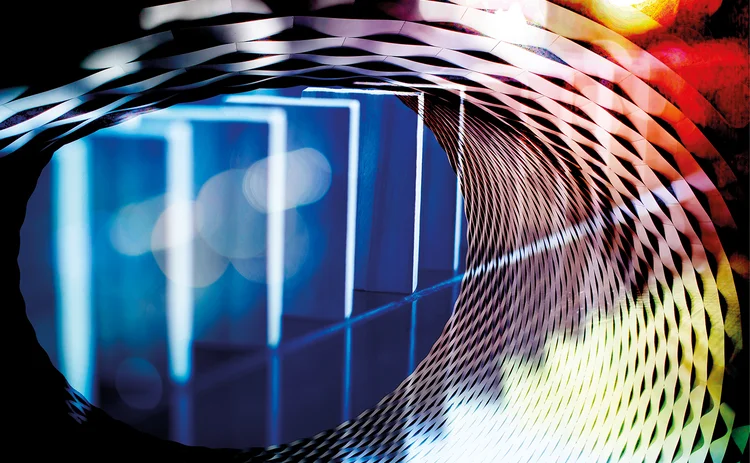
The Covid-19 pandemic has induced a kind of schizophrenia in loan-loss models. When the pandemic hit, banks overprovisioned for credit losses on the assumption that the economy would head south. But when government stimulus packages put wads of cash in the hands of consumers and businesses, the models changed course, leading banks to release reserves back into the income statement. This year, the models have shifted yet again as the stimulus programmes wind down and banks assess the impact on borrowers.
Banks have taken to manually adjusting the outputs to increase reserves. “The models are saying ‘release, release, release’ as we get out of the crisis,” says a credit risk executive at a large US regional bank. “The models are saying: ‘Given a good economic environment, we should be reducing reserves.’ But nobody’s comfortable with that level, given there’s still a lot of uncertainty in the economic environment.”
Banks are required to think ahead and set aside reserves to cover expected losses in their credit portfolios. Lenders outside of the US fall under the International Financial Reporting Standard (IFRS) 9, which divides loans into three groups. Performing loans are considered so-called stage 1 assets, assets that have significantly deteriorated in credit quality are classed as stage 2, and assets that have become impaired or lost value move into stage 3. Banks must hold enough provisions to cover 12 months of expected credit losses (ECLs) for stage 1 assets. For the other types of loans, they must hold reserves to cover the assets’ lifetime.
Under the Current Expected Credit Loss accounting standard in force in the US, the entire loan book is treated as if it contains only stage 2 or 3 assets. ECL provisions must therefore cover the life of the loans.
Deutsche Bank adjusted the outputs of its ECL models this year and last. During the first quarter of 2021, there were questions over consumers’ ability to repay loans as payment moratoria were eased. Therefore, the bank manually increased the provisions produced by its models. It did the same – and to a greater degree – in Q3 and Q4 2020 as the overall economic outlook remained hazy.
While the pandemic has thrown loan loss models into chaos, banks are forging ahead with machine learning algorithms that can replace many of the human tasks associated with credit underwriting. There is a catch, however: banks could leave themselves open to costly lawsuits if their models unwittingly discriminate against particular social groups. But, while the risks may be high, so too are the rewards.
With regulators on both sides of the Atlantic scrutinising the use of machine learning models, banks are adopting a ‘horses for courses’ approach, reserving the more advanced techniques for less sensitive tasks with lower explainability requirements. The more sensitive the application, the easier it must be to explain.
JP Morgan has been developing gradient-boosted decision tree models – where multiple decision trees are combined to reduce prediction error – to generate proprietary credit scores for use in its consumer and community bank. The machine learning models are able to take in hundreds of attributes. The bank says the models provide a finer-grained ordering of risk than traditional credit-scoring models that rely on logistic regressions.
Wells Fargo has developed methods for explaining a widely used form of deep neural network with rectified linear units. One such method decomposes the network into an equivalent set of local linear models that are easier to interpret, the bank says. If the technique proves successful, it may be an important step in helping lenders explain complex machine learning algorithms in credit underwriting.
Credit risk & modelling – Special report 2021
Read more
Sponsored content
Copyright Infopro Digital Limited. All rights reserved.
You may share this content using our article tools. Printing this content is for the sole use of the Authorised User (named subscriber), as outlined in our terms and conditions - https://www.infopro-insight.com/terms-conditions/insight-subscriptions/
If you would like to purchase additional rights please email info@risk.net
Copyright Infopro Digital Limited. All rights reserved.
You may share this content using our article tools. Copying this content is for the sole use of the Authorised User (named subscriber), as outlined in our terms and conditions - https://www.infopro-insight.com/terms-conditions/insight-subscriptions/
If you would like to purchase additional rights please email info@risk.net