
This article was paid for by a contributing third party.More Information.
Driving anti-money laundering efficiency gains using artificial intelligence
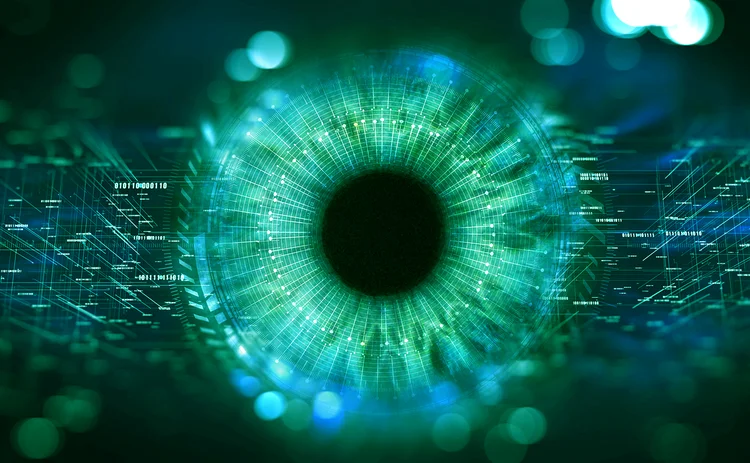
Anti-money laundering (AML) is expensive and labour-intensive, and artificial intelligence (AI) can offer improved efficiency gains. Could they be a match made in heaven? This Risk.net webinar, in association with NICE Actimize, took place amid the strain on banks’ back offices driven by the lockdown in response to the global Covid‑19 pandemic, and explores this potential pairing
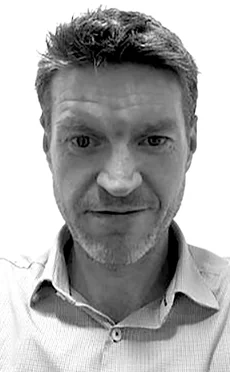
Today’s evolving regulatory environment and criminal typologies have influenced AML compliance teams to adopt AI technologies such as machine learning to improve detection and better focus analyst workloads.
The marriage of AI to existing compliance processes and risk modelling techniques has the potential to eliminate backlogs and create new efficiencies. But there may be some risks and question marks for those in the early stages of adoption.
The strain on many financial institutions has only increased in 2020 due to the unexpected arrival of Covid‑19. The subsequent lockdown presented enormous challenges. Fraud and AML teams are now – almost without exception – working from home, and the typical behaviour of corporate and retail customers has been inverted.
Problems around budgets, resources and priorities remain or have been redoubled for financial institutions. Regulators are still issuing fines for AML foul-ups. Financial criminals, meanwhile, are engaging in new rounds of scams and schemes to take advantage of the Covid‑19 pandemic.
“Clients are trading differently today than they were previously, and there’s a lot of volatility in the market,” said a chief compliance officer for a large European bank. “Most systems are calibrated to detect unusual activity, whether that’s increased volumes or changes in clients’ trading patterns. Consequently, there’s a lot more strain on our detection systems and our transaction monitoring guys are being kept very busy.”
The compliance head saw a rise in false positives, in part due to limitations on calibrating existing systems and the inability to anticipate current crisis event behaviours, warning that criminals and scammers are also ramping up activity.
“When you have a situation like Covid-19, the criminal fraternity see that as a great opportunity to try and test control frameworks within banks. We’ve seen an increase in the level of people who we would reject normally, and in any other situation they would not even attempt to open an account,” he said.
“The old classic scams, such as ‘I have $350 million tied up in a bank account in Nigeria; I just need this signature and some details’, had dissipated somewhat. Now, the scammers are back in force, using the pandemic situation to phish for an opportunity,” the chief compliance officer added.
Every crisis represents an opportunity for financial crime specialists looking to exploit the public, emphasised Andrew Fleming, global compliance MI, senior risk reporting manager at HSBC. This has led to a rise in scammers offering funds and posing as government or medical authorities to commit fraud.
“We’ve seen a number of these sorts of things already coming into play. One of my favourites was somebody in the US offering toothpaste that cured the disease,” said Fleming.
Covid‑19 could be a catalyst for creating more automation within banking processes, Fleming suggested, particularly if the illness or inability to work causes gaps in the ranks of teams working in risk and compliance roles.
“This pandemic could drive changes at a faster pace. It demonstrates the vulnerability of the human workforce and concentrates business minds on how they can derive value from the automation of processes, through robotic process automation and machine learning, and even deep learning, which is beginning to come into the fray,” he said.
“We’ve been looking at automation and driving digital change within the banking structure,” continued Fleming. “Machines might get the occasional virus that needs fixing but they’re not working from home and they work in a structure. Machines run 24 hours a day, seven days a week – and they’re driving efficiencies throughout the process.”
From a service provider’s perspective, Michael Barrett, senior director, product management, AML, at NICE Actimize, agreed that the pandemic has created an impetus for banks now that their focus is on operational efficiency and resilience.
“The need for technology to address some investigation elements is greater now than it ever has been before,” said Barrett. “Covid-19 has been a wake‑up call for many banking customers, some of whom had initiatives on the back burner to automate or streamline processes and address inefficiencies. Now they are in a situation where it’s front and centre of their agendas because they’re under pressure, and reliance on human interaction has become problematic.”
Seeing the wood for the trees
Big corporate customers for banks – such as retail department stores – are seeing an unprecedented drop in footfall in their premises since Covid‑19 lockdowns took effect. Some firms have gone into administration, while online shopping businesses are booming.
“Within that online demand you also get greater opportunity for financial crime,” Fleming said. “Fraudsters and money launderers are taking advantage of the situation because the volume of transactions is increasing, and companies may have a shortfall of staff because of sickness or be adapting to working remotely. Consequently, they are gambling that companies may miss fraudulent transactions that, under normal circumstances, would have been caught.”
Meanwhile, for money launderers, the Covid‑19 lockdown presents a different type of challenge because their schemes rely on cash-based businesses, such as barbers, tanning salons and car washes. “These criminals haven’t gone away. We still have drug dealers and fraudsters, and they need to get money into the system,” Fleming said.
“These people will look to use enablers, such as gatekeeper professionals, to get around systems. They may create new companies to try and demonstrate that they’ve got legitimate sources for funds. We’re going to be challenged with identifying these companies and identifying where this criminal money is now coming in,” he warned.
The chief compliance officer cautioned that the sudden changes in behaviour across society are making it harder – it’s increasingly difficult to spot the good money from the bad.
“Covid‑19 has triggered a massive change in behaviour across the board. We’re not a retail bank, so we’re more involved in combating the layering stage of money laundering, but we are seeing very strange behaviours from a number of our clients,” he said.
“That could be because they’ve started to launder money or it could just be a function of what’s going on in the market. We’re using up a lot more time and energy investigating those alerts than previously. It’s quite a challenge to see the wood for the trees,” he added.
Barrett proposed actions to address that challenge. First, by establishing what has gone wrong with the existing systems unable to cope with inverted transaction behaviour, and how these systems need to be refined.
“The first element to address is to perform some rapid tuning,” Barrett said. “We have an ActimizeWatch service, which allows us to monitor and understand where systems are degrading.”
“Where there’s a spike in alerts and unexpected behaviour, we’re able to model that with our own data science team on behalf of our customers, which can then provide the client with recommendations for thresholds to adjust. That’s a great way of us being able to quickly identify where a system needs to be refined,” he explained.
Opportunists among the criminals represent another challenge, Barrett suggested, requiring a focus on looking at new typologies and new behaviours. Suspicious behaviour patterns are emerging fast, and need to be found and captured quickly.
“In instances where cash-based industries have had no revenue for weeks or months, similar companies still operating a high turnover could be suspicious. Therefore, we’re working to analyse the potential for analytics based on behaviour by industry code as an example,” he said.
Of course, one danger with tweaking systems to detect unusual behaviours during crises is that, once normal conditions resume, a system could continue to identify trends that no longer apply.
“Absolutely, that’s a danger. We can’t model the new normal as the normal forever,” Barrett responded. “It will change again, and things will readapt and hopefully settle back down into a more consistent pattern. However, if current behaviour lasts for weeks or months, that’s too long to ignore. So we need to learn quickly now, then we need to adapt again once we are trending back into what would be the traditional normal pattern.”
Fleming agreed that model analysis element and dynamic risk assessment is exactly what’s needed. “We need to be able to develop new tools and strategies that are able to adapt in a dynamic risk situation. That includes being able to rapidly tune these technologies to meet the changing landscape,” he said.
Typical legacy systems at many banks lack the flexibility to be used dynamically, Fleming noted, taking too long to fix and change. “These new technologies need to be much quicker at adapting to new threats and new scenarios that come to the fore,” said Barrett. “I think every business has to be more agile in how it deals with threats. If we don’t do that then we’re going to get caught out.”
Approaches to machine learning
AML is just one of many applications for machine learning technology. Barrett gave his perspective on its use, beginning with the critical task of clustering and segmenting data, from which anomalies can be found, accurately targeting suspicious elements for any given data.
He described ‘step one’ for targeted detection towards getting better results from models: “We have machine learning models that assist with that clustering process, which carves out the data in the most effective way in the first place. Then we have additional AI technology that takes those segments and applies machine learning techniques to develop tuning thresholds and policies associated with those segments.”
He added: “Those technologies come hand in hand. Segmentation and optimised tuning are a package of machine learning we develop that allows for that automatic tuning based on precisely targeted segments,” he added.
The second step uses predictive models to learn the human investigation process, overlaying the human intelligence of the compliance team onto the systems themselves.
“Let’s learn about those investigations and factor that in as part of a model to feed back on any new alerts that have been produced. That way we can understand and provide insights into the likelihood of those alerts being particularly suspicious or, alternatively, even candidates for hibernation,” Barrett said.
“The third aspect is to provide machine learning models to introduce new detection capabilities themselves, by producing models for advanced anomaly detection, to allow for new typologies to be introduced, and so on,” he added.
Fleming added to this the need to demonstrate that internal controls are effective and the right governing structures are in place for delivering what’s needed for the customer, the business and the regulator. This means placing a focus on those controls and the supporting governance to ensure they are delivering what is required, he underlined.
“First of all, you want to be able to provide the customers with a seamless service that makes the end-to-end processing as painless as possible,” he said. “Second, you want to be able to protect the business and identify and reduce risks earlier on, driving operational efficiencies and effectiveness. We also want to be able to demonstrate to the regulators what we’re doing and how we’re managing risks effectively.”
New toys
Regulators have been somewhat neutral about AI and machine learning, in some instances encouraging innovation, but without specifying much in the way of good or bad practice – or what compliance benefits or pitfalls may await firms that innovate.
“Certainly, the UK regulator has never sanctioned any type of AI or machine learning products or processes,” said the chief compliance officer. “The regulator’s view is realistic; it’s that financial institutions should have systems and controls commensurate with the size and complexity of their business.
“If you tell them you’re processing a million payments a day, there is no way you can effectively screen them all without a sophisticated system that is well tested, checked and maintained. One of the few things regulators don’t mandate is whether any of your AML processes are automated. You could hire 10,000 people to do all your checks manually. You might go bust doing it, but you wouldn’t be in breach of the rules.”
On the other hand, the chief compliance officer noted regulatory dissatisfaction when, the regulators having conducted their investigations, they found out firms had invested in sophisticated technology but were then lax or incompetent in the testing, maintenance or use of it.
“Firms can shoot themselves in the foot,” he continued. “There are final notices mentioning when the regulator went into a certain firm they were disappointed to find that, although it had spent millions of pounds on various systems, the firm either hadn’t switched them on properly, or were just using the factory settings.”
Firms will get little sympathy from the regulator if they are investing in technology they don’t understand or don’t use properly, Fleming agreed. “The regulator is concerned that you understand you own the risk. Just because you’ve brought in a new toy – and no matter what the new technology is – they want to see it’s producing better results, and that you’re taking responsibility for it.”
Rare is the firm that chooses to build all its systems itself. Service providers therefore play an important role in providing regulatory confidence in their clients’ AML systems and models, and the governance in place to manage them responsibly.
“To meet governance expectations, evidence is provided on a number of different levels of detail right down to the specific algorithms used and the data attributes being fed in – everything around how the models are tuned to a specific customer environment,” said Barrett.
Transparency is a big part of this, he explained, requiring detailed dashboards to track model performance, allowing customers to peek under the bonnet and intervene when necessary.
Below-the-line testing strategies represent another key element to building regulators’ confidence for a new machine learning model, Barrett added. “Below-the-line testing is a key weapon in the armoury for ensuring that AML systems are strong, secure and doing exactly what they’re supposed to be doing,” he said.
“It’s vital there’s a solid below-the-line testing strategy in place. That’s so we can prove if all the thresholds are moved, or different rules are put in place, that these would be the events that would be produced, and if you investigated them you would understand that there is no suspicious behaviour there.”
Specialist skills
Part of that competence involves the skills of the AML teams tasked increasingly with managing technology as well as people. The skills required are inevitably shifting with the tools and techniques used.
Fleming said: “We have a good number of data scientists, but whether or not we have the right specialties in place to be good at working on the various types of financial crime, AML, fraud, tax evasion and tackling terrorism financing, I suspect not. You want to get the right people, with the right skill sets, and you do need to pay for that.”
The chief compliance officer agreed, adding that he had embarked on a part-time data science course. “Maybe I’m one of the compliance people that’s seen the light and is heading towards the data science field.” He suggested that, while there are probably enough data scientists on the payroll, AML specialist background experience is harder to come by and expensive.
“The population Venn diagram of those data scientists that cross over with any AML knowledge is very small, and that can put them beyond the budget that firms are willing to spend,” he said.
“Before you can get people to start committing budget to these things, you really have to make sure the organisation has made a commitment to being data-driven from the top. By that I mean having a chief executive officer and heads of compliance and risk who are tech-savvy themselves and understand the benefits.”
As a service provider, Barrett tries to supplement clients’ expertise with that of his own team, allowing them to focus their own resources on the crucial modelling efforts.
“We’ll build the infrastructure, we’ll flatten the data, we’ll provide the tooling. Our customers’ data science teams can then use that tooling to actually do the modelling,” he said. “We feel we can really help our customers have a laser-focused approach to doing modelling work and applying their data science minds in the right way.”
Listen to the full webinar, Using new AI tools to improve anti-money laundering and drive efficiency
The panellists were speaking in a personal capacity. The views expressed by the panel do not necessarily reflect or represent the views of their respective institutions.
Sponsored content
Copyright Infopro Digital Limited. All rights reserved.
As outlined in our terms and conditions, https://www.infopro-digital.com/terms-and-conditions/subscriptions/ (point 2.4), printing is limited to a single copy.
If you would like to purchase additional rights please email info@risk.net
Copyright Infopro Digital Limited. All rights reserved.
You may share this content using our article tools. As outlined in our terms and conditions, https://www.infopro-digital.com/terms-and-conditions/subscriptions/ (clause 2.4), an Authorised User may only make one copy of the materials for their own personal use. You must also comply with the restrictions in clause 2.5.
If you would like to purchase additional rights please email info@risk.net