
VAR lookbacks should shift dynamically, research suggests
Change-point analysis method helps identify regime shifts in equities markets, quants claim
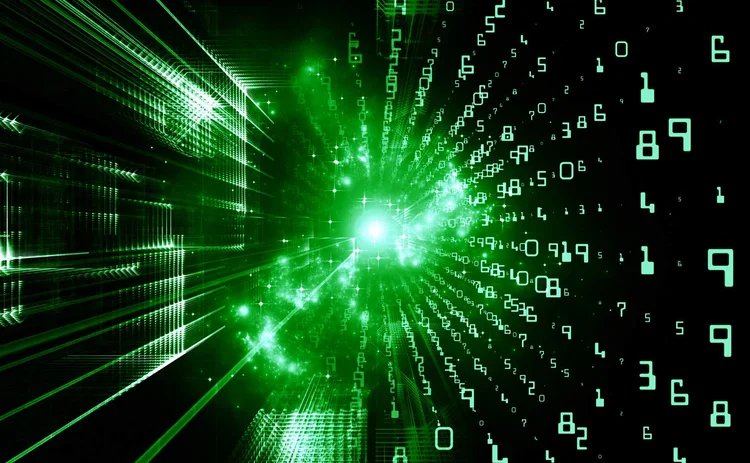
Calculating value-at-risk using historical data involves finding a way between two common pitfalls. If the window of historical data considered is too small, the result will be extremely volatile, as the dataset of daily data points will turn over rapidly. But if too long a lookback is used, the danger is it includes data that is no longer relevant, because it comes from a period in history that is too remote to be comparable to the present day.
Basel II mandated banks using their own models to set market risk capital to use a 10-day VAR model with a minimum one-year lookback, with the historical dataset updated at least every three months. Basel 2.5 allowed banks to use a shorter window, refreshed monthly, as long as it resulted in no reduction in capital – although it also backstopped this with a new stressed VAR add-on, a theme continued in the looming Fundamental Review of the Trading Book, which will upend the VAR approach completely. It also laid down that supervisors should approve and regularly review the windows used for different portfolios.
But new research suggests a much more flexible approach would work better. In a forthcoming paper to be published in the Journal of Risk Model Validation, Anri Smith and Chun-Kai Huang, of the University of Cape Town, demonstrate that different markets have different optimal window lengths, and the best length may also change over time for each – making the idea of a VAR model with a fixed window flawed, they argue.
Instead, they suggest using change-point analysis – a mathematical technique that relies on testing a dataset for homogeneity – to determine the longest suitable window for a given portfolio, gradually extending the size of the dataset until it fails the test.
Smith and Huang sampled 16 years of daily closing prices from six equity indexes – the Dow Jones Industrial Average, the FTSE 100, the Nikkei 225, the Johannesburg JSE/FTSE Top 40, the Sao Paulo IBOV index and the Bombay Stock Exchange 500. They calculated the daily VAR for each with 95% confidence, using windows of multiples of 50 days, up to 1,500 days, which equates to roughly six years’ worth of trading days.
They used three different commonly used VAR models: a parametric approach, in which VAR is estimated from a Gaussian distribution; a semi-parametric peaks-over-threshold method; and a non-parametric stationary bootstrapping method.
They then backtested each result in two different ways against the actual number of VAR exceptions: a Kupiec test, which compares the number of VAR violations with the expected number; and a Christoffersen test, which examines whether an exception is likely to be rapidly followed by other exceptions – a sign that the VAR model is performing badly.
The results showed that the optimal window size varied significantly from model to model and from index to index. Using the Kupiec and Christoffersen tests, an optimal result is one where the back test finds as many VAR exceptions as expected – one in 20, for a 95% confidence threshold – and where the exceptions fall randomly, rather than in ‘runs’. A run of exceptions implies some underlying cause the model has not taken into account.
For example, the optimal window for the Dow Jones was 500 days using a classical Gaussian VAR model, but 1,100 days under a model that used stationary bootstrapping; a 500–600 day window worked best for the FTSE 100 for all three approaches and both backtesting methods, and the best window size for the JSE Top 40 could be anything from 500 to 1,450 days, depending on the VAR model and backtesting technique used.
And there are other serious problems with having a fixed lookback, Smith and Huang add: “The models seem to be largely sensitive to window size selection. Although the optimal window sizes are determined as part of the process in this analysis, high-performance computing is required to record such results. There is also no universal choice for the best window size. These make the approach impractical in some applications. At the same time, these optimal window sizes may be restricted only to the time horizon in the current sets of data.”
The quants’ study uses change-point analysis to determine the longest suitable window for each index, gradually extending the size of the dataset until it fails the test
The quants’ study uses change-point analysis to determine the longest suitable window for each index, gradually extending the size of the dataset until it fails the test. This would indicate the dataset has expanded to include a change point, such as a sudden shift in macroeconomic conditions or a change in market rules.
Change-point analysis is relatively easy in computational terms – it doesn’t require high-performance computing support – and the VAR values produced from a window derived from change-point analysis stand up to backtesting much better than those produced from fixed-size windows.
The best results for VAR calculated for the Dow Jones index are derived using the Gaussian approach, according to the study’s findings, as measured using p-values.
“This is strong evidence that implementing a change-point procedure is more useful than a randomly chosen (or selected-by-convenience) fixed-window size,” write Huang and Smith. “More importantly, given the appropriate innovation assumption or treatment, the corresponding model can produce similar results as those arising from having previously identified the optimal fixed-window sizes. These make the proposed procedure practically more attractive.”
Only users who have a paid subscription or are part of a corporate subscription are able to print or copy content.
To access these options, along with all other subscription benefits, please contact info@risk.net or view our subscription options here: http://subscriptions.risk.net/subscribe
You are currently unable to print this content. Please contact info@risk.net to find out more.
You are currently unable to copy this content. Please contact info@risk.net to find out more.
Copyright Infopro Digital Limited. All rights reserved.
As outlined in our terms and conditions, https://www.infopro-digital.com/terms-and-conditions/subscriptions/ (point 2.4), printing is limited to a single copy.
If you would like to purchase additional rights please email info@risk.net
Copyright Infopro Digital Limited. All rights reserved.
You may share this content using our article tools. As outlined in our terms and conditions, https://www.infopro-digital.com/terms-and-conditions/subscriptions/ (clause 2.4), an Authorised User may only make one copy of the materials for their own personal use. You must also comply with the restrictions in clause 2.5.
If you would like to purchase additional rights please email info@risk.net
More on Risk management
To liquidity and beyond: new funding strategies for UK pensions and insurance
Prompted by policy shifts and macro events, pension funds and insurance firms are seeking alternative solutions around funding and liquidity
More cleared repo sponsors join Eurex ahead of cross-margining
End of TLTROs for banks and pension fund search for liquidity management tools drives uptake
Reimagining model risk management: new tools and approaches for a new era
A collaborative report by Chartis and Evalueserve on how the use of automation can combat the growing complexity of managing model risk due to regulation and market volatility
What Goldman’s appeal victory means for Fed stress tests
Decision could embolden more banks to appeal, analysts say. But others believe result is one-off
Clearing members rattled as CME approved to launch its own FCM
National Futures Association registration sharpens concerns about conflict of interest with CCP
CME files application for US Treasury and repo clearing
New entrant believes direct user access model will avoid accounting problem that hampers rival FICC
UST repo clearing: considerations for ‘done-away’ implementation
Citi’s Mariam Rafi sets out the drivers for sponsored and agent clearing of Treasury repo and reverse repo
Gensler to stick to Treasury clearing timetable
SEC chief promises to keep up the pressure for done-away trades