
This article was paid for by a contributing third party.More Information.

On the offensive – Seeking a new edge, buy-side invests in portfolio and risk analytics
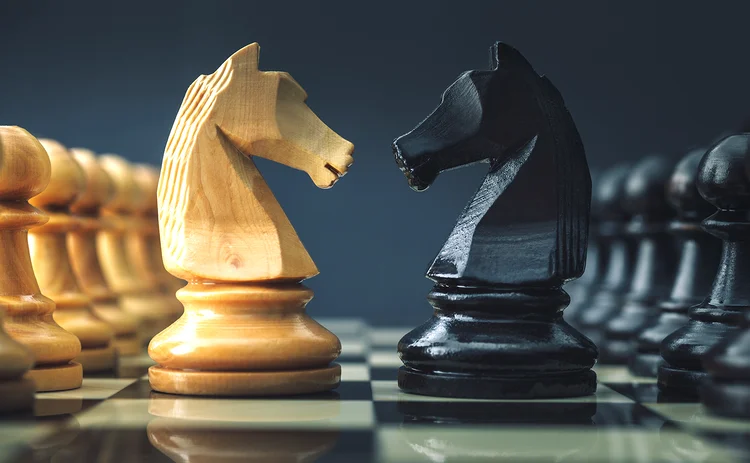
A fast-moving, headstrong hedge fund – hit by rare losses after a black swan event touched on an overweight country exposure – ponders adding fresh quantitative expertise. Much to traders’ chagrin, the chief investment officer and chief operating officer recruit several quants, running them through the gauntlet and backtesting their behavioural algorithms, before eventually deciding to take on the project themselves
Sometimes life imitates art, and this type of black swan scenario described above forms the plot of a recent episode of the US television series Billions. Though a stylised buy-versus-build scenario, real-life fund managers certainly empathise with it in 2018. Yield is tougher to find, and the investable universe has grown. The investment process is more creative and complex and – similar to the hedge fund portrayed in Billions – many investors are now evolving from traditional equity long-short to global macro, quant finance and beyond.
Where reality diverges from the series is in its treatment of risk. In Billions, the chief investment officer (CIO) serves almost as a black‑box risk function. The firm manages to adjust its country and sector exposure on a sixpence, usually with little internal discord, informational gaps or complexity in getting its orders filled. To large funds and certainly to institutional investors – who must carefully choreograph their entry into and out of new strategies, balance portfolio construction against their liabilities and manage an estate of ever-increasing data, modelling and analytics – that is a pipe dream, far removed from the intensive and deliberate processes that can take days or even weeks to complete without market impact.
Buy-side investment in risk technology today shapes this more subtle and sprawling drama. Whether modelling fixed income from Latin American or European periphery countries, forecasting potential effects of new benchmark rates post-Libor, completing transaction-cost analysis for hints of liquidity risk usable at pre-trade or anything in between, a robust risk management platform must be flexible. It must simultaneously present curves and heavy volumes of reference data – including unstructured, alternative data – consistently, make projects freely accessible to users who prefer different coding languages and development models, and provide a portfolio-level interface and view into underlying data elements that is customisable, reportable and auditable.
A new wave
Investors haven’t always supported an offensive technology approach to risk. Many investment managers and institutions have historically relied on their sell-side partners to provide value in this area and, excluding unavoidable costs such as market data provision, they have for the most part preferred to leave well enough alone. Following the 2008 financial crisis, that model began to shift as a natural consequence of the industry’s move to risk-adjusted returns and, as a rearguard action, both to protect against knock-on costs associated with sell-side capital charges and to manage regulation-driven market structure upheaval. Still, this boost was as much a matter of survival as it was of strategy. Much of the quantitative risk management work has remained separate from trading or portfolio management functions, usually sitting in Excel spreadsheets or siloed systems.
Taking this next step, a new wave of portfolio and risk technology investment now appears to be at hand. If the new operating model is ‘risk first then trade’, risk platforms must deliver data and capabilities to more pairs of eyes than ever before. Greenwich Associates, a leading industry consultancy, recently asked 54 buy-side investment managers to track where their operational budget is spent on technology and why.
Kevin McPartland, the author of the Greenwich Associates study, said the “biggest surprise” by far was the continued upward trajectory of risk and portfolio technology spend, including a two-thirds increase – an estimated $277 million – over the previous year. Firms are also wasting little time in taking advantage, with 77% reporting they are either already implementing risk and portfolio platform changes for the coming year or are currently evaluating a move.
Why is change coming now? Quite simply, targeting portfolio and risk analytics provides multiple avenues for amplified and scalable benefits across the investment management process. Tightening returns are driving more innovative thinking about strategies and allocation. A call to reduce operating and regulatory costs has prompted firms to seriously consider ways in which they can best deploy and preserve capital. Both objectives require the buy-side risk platform to do more.
The research by Greenwich Associates reveals a number of focus areas and activities – market and portfolio risk sit just above credit and rates, and scenario analysis and stress testing are joined by pre‑trade analytics around Greeks and sensitivities. But the themes that came out on top are customisation capability – around data analysis and end-reporting alike – and integration. As McPartland points out, the challenge isn’t a matter of millisecond speed for most buy-side firms, rather it is one of achieving clarity and catalysation. So perhaps the more fundamental issue is a step further back: the largest respondent group – more than half of those surveyed – said that change is required to simply eliminate historically bound, manual processes. Making this change is step one in achieving a more holistic and actionable view into risk that they’ve never had – and now need.
Selective strategies
Firms will always start at a point of universal consensus. Extraordinary results can certainly harness institutional attention: making a five-figure saving on a single trade, saving millions of dollars on Solvency II-required regulatory capital for an insurer or effectively entering a greenfield market, for example.
To illustrate, take a pertinent and particularly resilient asset class in recent years – emerging market (EM) debt. Major index data showed returns as high as 13% on sovereign debt issuance in 2017. After demand and issuances further heated up in early 2018, actively managed, local-currency-denominated EM bond funds had brought back a 4.4% return in less than a month, according to BlackRock data. From a pensions investment committee filling out a liability-driven strategy, to a hedge fund exploring global macro, to an asset manager looking to expand its product offerings, this slice of global fixed income is ripe with attractive and often mispriced offerings, generating additional yield.
Risk teams find it difficult to select the right opportunity and then construct the risk tolerance and, ultimately, the allocation around it. Trade selection and portfolio optimisation begins at a bedrock level, with comprehensive and up-to-date valuation analytics in place, including conventions, reference data, pricing history for related derivatives, forward curves and bond definitions. Of course, this deluge should allow users to flexibly model the pricing of the bond and the proper size of the order, and fill in informational gaps. But it should also provide historical context around potential illiquidity, and highlight performance fluctuations caused by external factors such as political risk.
With a holistic picture of the debt instrument in place, the buy side can move on to more practical trading matters – how to properly hedge the credit risk and, if the bond is local-currency-dominated, the foreign exchange risk – and locating the most stable sources of liquidity if instability strikes. Likewise, the firm should be able to predict the risk premium and the lifetime cost of executing the trade, including optimised clearing and collateralisation, and be able to compare those computations against potential simulacra – for example, investing in an actively managed fund rather than a directly managed one.
Finally, contextual analysis is performed on the portfolio by assessing attributes of the debt that may overlap with current holdings. Even if the EM is new to the firm, it may possess hidden similarities – national dependence on similar industries or commodities, regional or geopolitical entanglements, or even vulnerability to natural phenomena – that cross over. Extensive backtesting and measuring the probability of this compounded portfolio risk, and calibrating and recalibrating exposures and concentrations accordingly, means schemata must be developed and layered in to allow users to slice and dice portfolio holdings’ underlying risk elements.
It is true that playing in EM sovereign borrowing is more art than science.
A measured and selective posture is required, even when the current temptation is ‘all in’. Doing this well demands an analytics infrastructure that can be spun up fast, and can readily link quantitative thinking, trading operations and portfolio managers – a central hub that manages the construction of the investment process from end to end. In this setting, manual processes are a recipe for cross‑functional tension, operational lag and even disaster. And the same stakes apply in putting securitised debt or more exotic derivatives to work – only with added quantitative complexity.
Marked territory, new spaces
If the first step in evolving the investment process is ‘what?’, the technological questions follow with ‘how?’ Customisation was the top priority for the respondents of the Greenwich Associates survey. Choosing a new risk platform was the next highest priority, with 65% of votes – but, with 63%, the ability to integrate with the existing stack came a close second. This speaks to a recognition that the data, coding and modelling stores will not change overnight; indeed, if done right, optimal platform flexibility should simply allow them to become more accessible, rather than re-engineered and replaced. The main challenge in this area is territorial tension: the function and preferences of the users – risk managers, quants, traders, portfolio managers and technologists – who must all be involved in the build-out.
It’s often a question of style. In recent years, more quantitative work has moved to Python, the coding language valued for its ability to use microservices architecture (MSA) to create newly developed risk functionality – a particular portfolio optimisation tool, or other building blocks that can stand on their own, for example. In addition to being independently developed and self-contained – allowing rapid customisation and completion – microservices can also interface directly with disparate static, modelling and reporting data stores to decrease heavy run times. MSA has become an especially popular method for derivatives projects as it has become the subject of more internal analysis and regulatory reporting.
By contrast, services-oriented architecture is the favoured model for software development among trading technology teams. This more traditional approach relies on componentry and defined processes to complete tasks, emphasising communication via application programmable interfaces and the ability to wrap institutional-grade support, such as cyber security and business continuity, around it.
Buy-side firms today are learning that an effective risk analytics platform is able to speak to both sides of this divide, by being architecture-agnostic. Technology should provide a space where quants can write a microservice and freely publish to the platform, after which the tech team can seamlessly enable wider enterprise integration. The end goal is ultimately to reduce developer duplication and recoding, which should lend more time to value-added activities and reduce operational risk.
Readiness is everything
To realise the advantages of a risk-weighted world, they are now building a risk foundation that is flexible, open to integration and customisable.
To some, that may sound like a ‘Goldilocks proposition’, but in 2018 it is no fairy tale. Increasingly, if your firm isn’t aspiring to a higher level of sophistication, it is already behind its peers.
The author
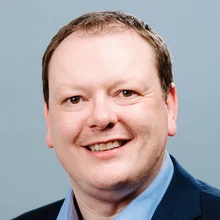
James Church, Vice President, Product and R&D, FINCAD
James Church is responsible for the strategic direction of all FINCAD products and has more than 15 years of experience in the software industry. Before joining FINCAD, he was vice president of OLAP Product Management at Business Objects and, prior to that, director of OLAP Product Management at Crystal Decisions.
About FINCAD
FINCAD is the leading provider of enterprise portfolio and risk analytics for multi-asset derivatives and fixed income. An industry standard since 1990, our advanced analytics, flexible architecture and patented technology enable financial institutions to make better investment and risk decisions. Our goal is to provide our clients with solutions that help them achieve their goals, with no compromises. Clients include leading global asset managers, hedge funds, insurance companies, pension funds, banks and auditors.
For more information, visit www.fincad.com
Download a copy of the Greenwich Associates report, Developments in Buy-Side Risk Technology
Sponsored content
Copyright Infopro Digital Limited. All rights reserved.
As outlined in our terms and conditions, https://www.infopro-digital.com/terms-and-conditions/subscriptions/ (point 2.4), printing is limited to a single copy.
If you would like to purchase additional rights please email info@risk.net
Copyright Infopro Digital Limited. All rights reserved.
You may share this content using our article tools. As outlined in our terms and conditions, https://www.infopro-digital.com/terms-and-conditions/subscriptions/ (clause 2.4), an Authorised User may only make one copy of the materials for their own personal use. You must also comply with the restrictions in clause 2.5.
If you would like to purchase additional rights please email info@risk.net