Journal of Risk Model Validation
ISSN:
1753-9579 (print)
1753-9587 (online)
Editor-in-chief: Steve Satchell
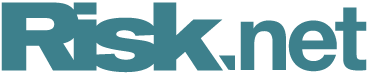
Shapley values as an interpretability technique in credit scoring
Hendrik Andries du Toit, Willem Daniël Schutte and Helgard Raubenheimer
Need to know
- Shapley value is introduced as a machine learning interpretability technique in credit scoring and the effectiveness of this technique is tested on various simulated datasets.
- A traditional model as well as machine learning algorithms are used to generate the Shapley values.
- The Shapley value results are compared to a metric called the Weights of Evidence (WOE) value.
- The results show that Shapley values are a related measure to WOE.
Abstract
The use of machine learning algorithms in credit scoring can be enhanced by an improved understanding of the reasoning behind model decisions. Although machine learning algorithms are widely regarded as highly accurate, their use in settings that require an explanation of model decisions has been limited due to a lack of transparency. This is particularly the case in the banking sector, where the model risk frameworks of banks frequently require a significant level of model interpretability. In this paper, the Shapley value is evaluated as a machine learning interpretability technique in credit scoring. The Shapley value is a model-agnostic machine learning interpretability technique that quantifies the contribution of each feature in the prediction of a specific observation. The effectiveness of this technique is tested on various simulated data sets with covariates from different underlying distributions that are linearly and nonlinearly related to the outcome. Traditional models (eg, logistic and linear regression) and machine learning algorithms are trained on the data and the Shapley values are generated. Our results show that Shapley values are related to weights of evidence (a well-known measure in the scorecard literature) and can be used to explain the direction of relationships between explanatory variables and the outcome.
Copyright Infopro Digital Limited. All rights reserved.
As outlined in our terms and conditions, https://www.infopro-digital.com/terms-and-conditions/subscriptions/ (point 2.4), printing is limited to a single copy.
If you would like to purchase additional rights please email info@risk.net
Copyright Infopro Digital Limited. All rights reserved.
You may share this content using our article tools. As outlined in our terms and conditions, https://www.infopro-digital.com/terms-and-conditions/subscriptions/ (clause 2.4), an Authorised User may only make one copy of the materials for their own personal use. You must also comply with the restrictions in clause 2.5.
If you would like to purchase additional rights please email info@risk.net