
This article was paid for by a contributing third party.More Information.
From chaos to clarity: the role of clean data in banks’ digital journeys
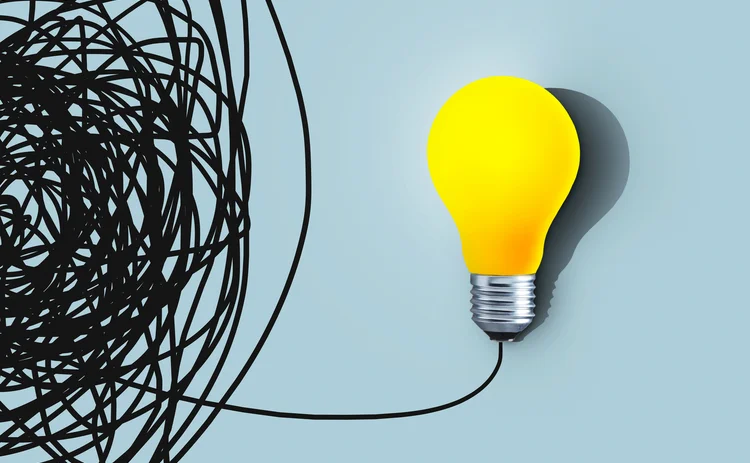
In a Risk Live Europe panel session sponsored by Numerix, experts explored the role that clean and accurate data plays in digital transformation. Here we examine the main themes arising from the discussion
The panel
Sarthak Shreya, Product manager, Numerix
Marina Antoniou, Board member, Institute of Chartered Accountants in England and Wales (ICAEW) Financial Services Faculty
Aiman El-Ramly, Chief business officer, Zema Global Data Corporation
Mikael Sörböen, Head of risk systems, chief information officer (CIO) risk markets, BNP Paribas
Deenar Toraskar, Risk chief technology officer (CTO) architect, UBS
Architecture options
Digitalisation has transformed the financial services sector over the past decade. Yet, with the rapid pace of change and continual technological advances, it can sometimes feel like a never-ending journey.
A fundamental decision for banks is to choose the most appropriate architecture model for their business. “Banks need to ask themselves whether they can continue to sustain on-premise into the future,” said Aiman El-Ramly, chief business officer at Zema Global Data Corporation. “If not, are they going to move to a public cloud or to a provider with a private cloud? Each of these choices has different support challenges.”
Other factors must be considered too, such as culture, legacy, cost, speed and the operational resilience of third-party providers, particularly in light of the European Union’s Digital Operational Resilience Act.

Sarthak Shreya, product manager at Numerix, added: “The choice also depends on the use case. For example, XVA [valuation adjustment] requires deep domain expertise and significant compute power, along with data traceability and lineage. To ensure this is done in a secure, operationally efficient manner, it makes sense to use a vendor with specialist expertise. But, on the flipside, for sensitive customer information, it may be a better option to remain in-house or on a private cloud.”
Marina Antoniou, board member at the ICAEW Financial Services Faculty, also said: “It is vital for organisations to invest in scalable systems and infrastructure to be able to efficiently absorb and process the huge influx of unstructured data, particularly when it comes to artificial intelligence [AI] and large language models [LLMs].”
Artificial intelligence
AI is changing the data landscape, Antoniou explained, but “it is not going to make traditional data mining obsolete. It complements this practice by offering more powerful tools to enable time series analytics.”
However, AI is not without its challenges. Panellists raised the issues of ethics, responsibility, explainability, the increased risks of synthetic fraud using generative AI, and the difficulties of combining teams with the relevant expertise in data, machine learning and risk.
Deenar Toraskar, risk CTO architect at UBS, said firms need to look beyond the hype around AI: “People are using LLMs where simpler models could work. Why spend 10 times the energy and cost to send a query to an LLM, when a simple rules-based algorithm would suffice?”
Shreya concurred: “From a time series point of view, machine learning-oriented statistical approaches can be easier to use, but even simple approaches such as EWMA [exponentially weighted moving average] or Garch [generalised autoregressive conditional heteroscedasticity] are still very relevant. These models can be applied to fill historical gaps and produce consistent and complete time series data, which is what Numerix clients are really looking for.”
Defining clean and accurate data
With regard to digital transformation, both the panel and the audience had far more concerns about poor data quality than data technology. However, clean and accurate data can be defined in many ways. In addition to the processes vendor data may need to undergo, Antoniou expressed concern about issues such as data bias and the availability of robust data, which have been a major challenge within environmental, social and governance.
Shreya underlined the challenges of measuring data quality. “If there are poor model outputs, it is likely that the input data was also poor, but it is difficult to look at data objectively and identify good versus bad,” he said.
He also explained the best way for banks to proceed with their data challenges: “It is first important to define the problem that needs to be solved. For example, ensuring all equity swaps or discount factor curves are consistent across systems. It is also important to have a road map and take things one step at a time, rather than expecting everything to be clean and perfect from the get-go.”
Responsibility, culture and governance
Mikael Sörböen, head of risk systems, CIO risk markets at BNP Paribas, highlighted the issues of responsibility and culture. “For many years, our experience was that every time data was missing or inaccurate, it was labelled an IT problem. We were successful in demonstrating that maybe 10% were IT problems, but 90% were either process or data ownership problems. So, for me, culture is key. If we are serious about data quality, everyone needs to take responsibility. If risk, finance or IT are seen as second-class groups of people that can waste their time chasing up data-quality issues that derived from the front office, you are never going to get good-quality data.”
Antoniou agreed: “Culture comes from the top. You need leadership buy-in and an integrated approach across the organisation. Decisions around data quality and technology should not be looked at separately – they should be considered as part of an overall digital strategy and framework. Key governance forums need to be in place to monitor any breaches and to escalate any problems. So if something goes wrong with the data, it is not just a technology problem.”
Shreya added: “Any governance framework should ensure there are manual steps, incident management procedures and fallback mechanisms in place when needed.”
Self-service data infrastructure
In addition to culture and governance, Toraskar highlighted the importance of data autonomy. “Self-service, cloud-based data infrastructure with complete visibility means there is no reliance on IT to determine what is wrong. This means democratising access to data, allowing users to identify data owners and drill down into the data if they find an issue with quality. To execute this, you need efficient catalogues, intuitive user interfaces and collaboration features. If that is done at scale within an organisation, it will reduce data quality and silo issues.”
In summary
Data quality is a pre-eminent concern when it comes to digital transformation. In addition to overcoming architectural hurdles, banks must ensure the fundamental building blocks for clean and accurate data are in place. This includes strong governance frameworks, along with organisational culture and clear responsibility.
Sponsored content
Copyright Infopro Digital Limited. All rights reserved.
As outlined in our terms and conditions, https://www.infopro-digital.com/terms-and-conditions/subscriptions/ (point 2.4), printing is limited to a single copy.
If you would like to purchase additional rights please email info@risk.net
Copyright Infopro Digital Limited. All rights reserved.
You may share this content using our article tools. As outlined in our terms and conditions, https://www.infopro-digital.com/terms-and-conditions/subscriptions/ (clause 2.4), an Authorised User may only make one copy of the materials for their own personal use. You must also comply with the restrictions in clause 2.5.
If you would like to purchase additional rights please email info@risk.net