
This article was paid for by a contributing third party.More Information.
Leading-edge LLM approaches: predictive analytics for M&A targets
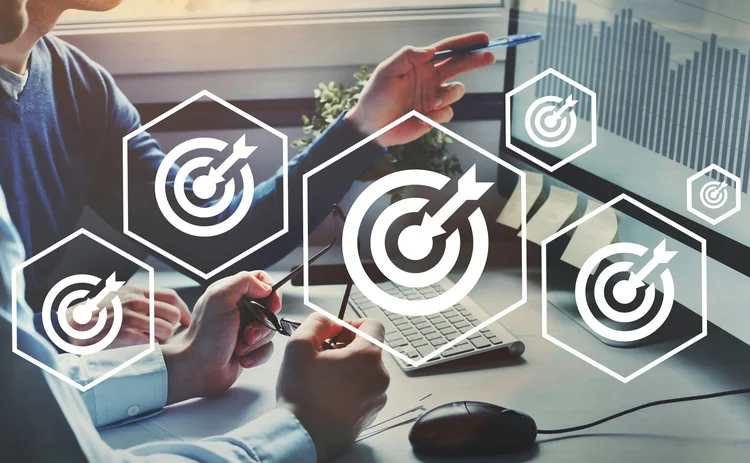
Utilising large language models (LLMs), London Stock Exchange Group’s (LSEG’s) StarMine Mergers and Acquisitions Target Model is transforming the landscape for mergers and acquisitions (M&A) target predictions. LSEG’s Nelson Chin and Richard Goldman discuss the company’s ongoing innovation in data analytics
Capital markets are undergoing a transformation driven by the adoption of LLMs, a type of artificial intelligence (AI) capable of processing and understanding natural language. LLMs are revolutionising the way firms analyse data, identify trends and make informed decisions. LSEG’s data science teams and quantitative analysts worldwide use LLMs in various applications, showcasing their versatility and impact.
In August, LSEG’s StarMine team launched its latest model to utilise LLMs, predicting M&A targets with more precision than ever. LSEG discusses the challenges for firms in securing insights from unstructured data, the key considerations for LSEG in ensuring accuracy of information and what’s next for the models.
Inspiration for the model: navigating a complex data landscape

The StarMine M&A Target Model uses an LLM – Bidirectional Encoder Representations from Transformers (BERT) for natural language processing (NLP) using Reuters News Archive (RNA) – known as BERT‑RNA. The model ranks potential M&A targets for more than 38,000 public companies globally over the following 12 months. Nelson Chin, director, data science and engineering at LSEG Data & Analytics, explains how BERT‑RNA – LSEG’s financial domain-specific variant of BERT and Google’s open-source technique for NLP pretraining – is pretrained on RNA data. “We fine-tuned BERT‑RNA using historical M&A events to enhance the model’s ability to predict the likelihood of a company becoming an M&A target,” says Chin. It analyses news articles from the previous six months, providing a company-level score based on relevant sections extracted from aggregated document-level estimation.
The creation of the StarMine M&A Target Model was inspired by the challenges clients faced in creating robust models. Richard Goldman, global head of sales strategy and execution, quantitative analytics at LSEG, noted that the StarMine team – with more than two decades of expertise and access to LSEG financial data – played a pivotal role in evaluating datasets, providing clients with a benchmark for using LLMs and unstructured data effectively. “Even firms with sophisticated in-house capabilities find advantages in using StarMine’s models,” he says.
Chin notes that the model’s initial focus was on digitising investment banking, seeking to extract valuable insights from diverse, fragmented and unstructured data. “The primary objective was to employ predictive tools to uncover potential M&A opportunities and facilitate the origination of new deals,” he explains. Beyond investment banking, the model extends its benefits to risk mitigation, avoiding short positions in companies likely to be acquired. Additionally, it serves quant use cases by enhancing multifactor alpha and risk models with an orthogonal signal.
Chin says the StarMine M&A Target Model has ongoing plans for enhancements. “We are considering extending coverage to private companies and exploring additional data sources such as earnings conference call transcripts, Securities and Exchange Commission filings and broker research reports, which would provide expanded orthogonal signals. Future enhancements may also include expanding the model to cover non‑English language news articles.”
Ensuring accuracy: key considerations
The StarMine M&A Target Model relies significantly on the quality of input data, and Chin stresses that this a key differentiator. “Leveraging LSEG’s extensive point‑in‑time data – particularly the rich M&A history in the deals database – is crucial for training,” he says. Challenges in labelling the Reuters news corpus are addressed through selective inclusion of relevant articles, exclusion of unrelated content and a focus on the initial release for accurate training. The Reuters newsfeed plays a vital role in ensuring accuracy by distinguishing new stories from updates.

Goldman emphasises the importance of accurate and unbiased data: “Reuters’ intense focus on providing unbiased news – along with the historical database being a point-in-time source – provides a reliable foundation, avoiding editorial and forward-looking biases.”
Data augmentation – or a process called ‘named entity replacement’ – is employed to avoid overfitting the model, ensuring individuals’ and companies’ names and locations are anonymised. This step prevents the model from associating a mere mention of a specific company with prior M&A events, contributing to a more generalised and accurate outcome. This anonymisation extends to identifiers such as tickers and Reuters Instrument Codes. Addressing the challenge posed by news articles mentioning multiple companies, the team parses and preprocesses articles to attribute each subarticle to the correct company. This enhances the precision of the model, making it more reliable in predicting M&A targets.
Another crucial consideration is symbology resolution. Especially when dealing with historical data, complexities arise because many databases reflect current knowledge, not the historical context required for training models. Goldman explains that going back in time requires understanding what each symbol meant at that specific historical moment – whether a Committee on Uniform Securities Identification Procedures (CUSIP) or International Securities Identification Numbering (ISIN) code, a ticker or company name. “For example,” he says, “if a database references the ticker ‘C’, today it signifies Citigroup, but in 1990, it represented Chrysler.”
At LSEG, input from RNA is leveraged, using the Reuters Instrument Code for preprocessing to extract company-specific sections. However, the model also incorporates a fundamental component that utilises other identifiers. Chin advised that, to handle this complexity, the team relies on the LSEG Quantitative Analytics Database, which excels at point-in-time symbology resolution. “This enables us to seamlessly align multiple content sets for a particular company, simplifying a typically complex problem,” he says.
Furthermore, the team uses combined models, which integrate an LLM to analyse unstructured data, such as news, and traditional structured data, including financial statements, corporate actions, credit quality and deal information. This approach, which is central to the M&A Target Model, synergises insights from diverse sources. Goldman suggests the key is to create a synergy that enhances the accuracy of predictions. “A company with weaker financials might be a likely target due to specific circumstances revealed in news,” he says.
Chin says this approach offers advantages over competitors that primarily rely on fundamental data. Unstructured data, comprising faster-moving signals, allows more timely updates – especially with reliable 24/7 breaking news coverage. “The major benefit of combined models lies in expanding the coverage universe, meaning more companies receive a score,” says Chin. Users have access to combined and individual scores for the fundamental and text components, providing a comprehensive and adaptable solution.
Beyond M&A: exploring diverse applications of LLMs
The StarMine team is exploring the extended applications of LLMs, moving beyond predicting M&A targets. One avenue involves utilising LLMs for predicting financial events such as bankruptcies and defaults. Ongoing research is focused on enhancing the performance of the Text Mining Credit Risk Model by incorporating LLMs.
More widely, LSEG has been exploring the use of LLMs for four years, initially with BERT and, more recently, with generative LLMs such as ChatGPT.
Goldman says LSEG’s long-term strategic partnership with Microsoft has facilitated collaboration on leveraging its Microsoft Fabric technology and building LSEG’s next-generation data platform. “We are also taking advantage of their experience with generative AI,” he adds.
Goldman notes that LSEG is using LLMs for a variety of purposes, ranging from improving internal productivity to improving the customer experience. One example he highlights is the application of LLMs and machine learning models at LSEG’s Institutional Brokers’ Estimate System (I/B/E/S) team for enhancing the verification process for estimates obtained from analysts’ reports. He notes: “By leveraging AI, we have significantly reduced the time to transition from preliminary estimates to verified estimates by 15%, ensuring more accurate and timely information in the data collection process.”
Another example is a team of data scientists in Edinburgh focused on voice-to-text initiatives, creating live transcripts of company conference calls using LLMs. These accurate transcripts generate real-time text feeds, providing valuable metadata for data science and quantitative analysis, offering insights beyond simple transcripts. “Additional information, such as identified topics, enhance the feed’s utility for various use cases,” says Goldman.
LSEG is working on a transcript sentiment database, enabling granular analysis of speakers, topics and sentiments, sentence by sentence. “This further illustrates how we employ LLMs for effective data collection, enabling our clients to gain more insight from transcripts,” says Goldman.
LSEG is also developing a co-pilot tool to make user interaction more intuitive. By utilising generative AI, Copilot eliminates the need for coding and simplifies access to LSEG’s vast data repositories. With more than 60 content sets, each containing terabytes of data, Goldman explains that the goal is to make this wealth of information more easily accessible to a wider audience. “We are integrating this process into our desktop solutions in collaboration with Microsoft.”
Conclusion
As LSEG’s exploration of LLMs progresses, the StarMine M&A Target Model reflects the expertise, technology and innovative solutions of its data science teams. Inspired by the challenges faced by clients navigating complex data landscapes, this model has become a benchmark for leveraging LLMs to evaluate datasets effectively.
The ongoing commitment to accuracy, demonstrated by thorough attention to data quality and symbology resolution, positions LSEG as a leader in harnessing the potential of LLMs. The exploration of diverse applications and collaborations with industry leaders underscores the impact LSEG has on shaping the future of data-driven financial insights.
Sponsored content
Copyright Infopro Digital Limited. All rights reserved.
As outlined in our terms and conditions, https://www.infopro-digital.com/terms-and-conditions/subscriptions/ (point 2.4), printing is limited to a single copy.
If you would like to purchase additional rights please email info@risk.net
Copyright Infopro Digital Limited. All rights reserved.
You may share this content using our article tools. As outlined in our terms and conditions, https://www.infopro-digital.com/terms-and-conditions/subscriptions/ (clause 2.4), an Authorised User may only make one copy of the materials for their own personal use. You must also comply with the restrictions in clause 2.5.
If you would like to purchase additional rights please email info@risk.net