
This article was paid for by a contributing third party.More Information.
Investing in operational readiness to optimise FRTB capital
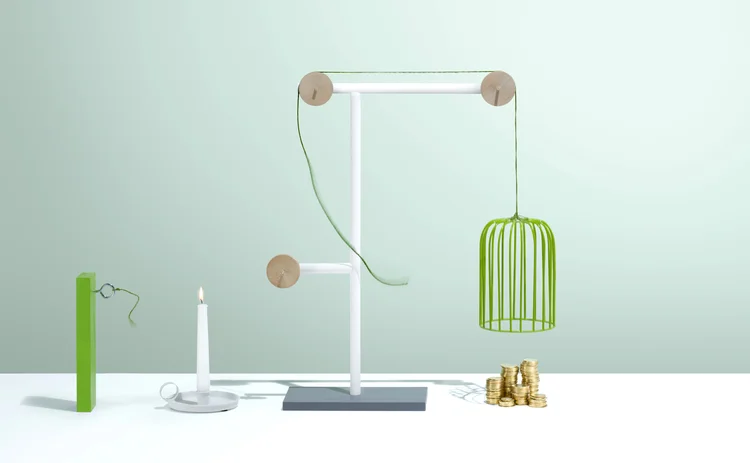
A panel of industry experts discusses the implementation of the Fundamental Review of the Trading Book (FRTB), the burden of investment into data and infrastructure for FRTB compliance, the considerations for banks in using the standardised approach (SA) and the internal model approach (IMA), and how vendors are adapting solutions to weigh up the capital efficiencies of both, as well as strategies and tools for profit-and-loss attribution (PLA) and risk factor eligibility tests (RFETs)
The panel
- Anastasia Polyakova, CQF, Product manager, market risk, ActiveViam
- Hany Farag, Senior director and head of risk methodology and analytics, CIBC
- Anna Holten Møller, Senior analyst, market risk models, Nykredit
- Manoj Rathi, FRM FCS, Senior functional analyst, market risk, Cleared Europe Services
- David Rogers, FRTB subject matter expert, Bank of America
How has uptake of market risk models been evolving in the European market and beyond, and how are vendors positioning themselves to support banks in navigating the new regulatory requirements?
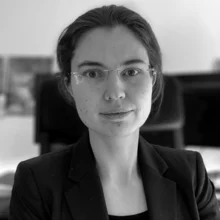
Anastasia Polyakova, ActiveViam: We are hearing that uptake of the IMA is not as high as first expected. Still, banks would like to assess the viability of the IMA to determine if the capital savings it would provide justify the added complexity. Other banks will implement the SA without considering the IMA, or implement the SA first and evaluate the viability of the IMA desk-by-desk. Even those not considering implementing the IMA would like to be able to compare the capital requirements from both methodologies.
ActiveViam's ability to run both the IMA and SA on the same platform allows banks to determine what approach to use for each of their desks, with a limited increase in operational expense. They will also benefit from a step-by-step walkthrough of the calculations to support reconciliation and reach consensus interpretation, as well as reporting to multiple regulators through multi-jurisdictional support.
Hany Farag, CIBC: My observation is that the uptake is gradually increasing, particularly among the large banks – perhaps up to 50%. Capital smoothness is more easily achieved by employing the IMA, as the SA can have some cliff effects and counterintuitive aspects to it. The IMA is certainly more risk-sensitive. Vendors are improving their products, particularly in the data space, though, in my opinion, not many are up to speed yet with meaningful offerings. Their rate of progress suggests they may be gradually building their products with a 2025 timeline.
Manoj Rathi, Cleared Europe Services: The FRTB regulations have led to a significant uptake of market risk models, and banks have increased their focus on building the systems and technology to handle the complex requirements. Vendors are providing cloud-based offerings, as well as assistance in building data capabilities, front-to-back integration, enhanced analytics and trade-level granularity. This helps banks optimise and leverage their risk capabilities, derive more real-time insights, and assess the impact of trades on overall market risk charges to, ultimately, take more informed trading positions.
David Rogers, Bank of America: For the majority of banks, the primary focus has been on implementing the SA within Europe to be compliant with the regulatory timeline for both reporting and capital. Priorities are now shifting to rolling out SA implementation for other jurisdictions, with minor tweaks designed to navigate any local regulatory divergences. Banks have spent considerable effort developing their IMA capabilities in parallel, and those programmes are seeing significant acceleration in 2023. With European implementations already in place and moving into business as usual – at least on the SA side – the primary demand is for more sophisticated analysis suites, generating what-if scenarios and explaining analysis capabilities to facilitate senior management decision-making around IMA versus SA, risk representation, desk structures and the trading book/banking book boundary.
What are the key considerations for banks deciding between the IMA or the regulator-set SA for calculating market risk capital requirements, and what are the trade-offs involved in these decisions?
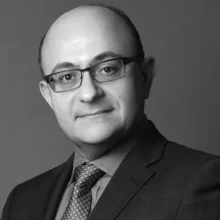
Hany Farag: There is substantial work required to improve and better align risk and front-office systems. This is highly nontrivial and is a multiyear project. The introduction of the PLA test requirement has made it necessary for risk management systems to include considerable sophistication and computational capability to mimic front-office pricing while producing all the required risk measures. Additionally, work on non-modellable risk factors (NMRFs) is rather demanding, and the statistical tests imposed by the regulations to reduce this capital are difficult to pass. This substantial effort seems to discourage some banks from pursuing the IMA, as they cannot determine the specific impact of these tests a priori. Finally, data requirements are also quite significant, whether for reducing the impact of NMRFs or enhancing the historical data with new sets of risk factors that are typically required to achieve alignment between risk management and the front office. On the other hand, successful implementation of the IMA should allow banks to have more predictable and smoother capital, more risk control and transparency, and a much more efficient capital level.
Anna Holten Møller, Nykredit: The main consideration is whether there is a business case for using internal models. For some banks there will not be a business case, either due to capital floors, NMRF challenges or simply because they would be highly unlikely to pass the PLA tests because of the nature of the products in the portfolio. For there to be a business case, these banks would need to make changes to their core businesses, which they are unlikely to do.
The regulators have a wild card as to whether they will require certain large banks to use internal models. However, that would undermine the SA model as a credible fallback. Even if banks do not implement the IMA, FRTB has increased the focus on risk factors, the PLA test and capital management generally. In the past, traders may not have paid a lot of attention to their capital use but focused primarily on risk and profit and loss (P&L). Capital has now become a big focus area, with a lot of involvement from traders and the front office, which is, in my opinion, good for capital models.
Anastasia Polyakova: Historically, most banks already run an IMA-like model for market risk, such as simulation-based methodologies for value-at-risk and expected shortfall. This is their starting point for IMA reporting.
However, uplifting those existing models to comply with FRTB rules is a huge investment, and there are additional running costs related to market data acquisition to perform RFETs. Capital relief potentially delivered by the IMA, which is limited by the output floor, might not bring the desired return on investment.
FRTB model eligibility often requires that risk and P&L pricing models converge, and risk factor treatment is scrutinised to account for liquidity and market data observability. To pass the RFET, banks need to obtain market data to prove the observability of prices, which is a big issue in some regions. While capital savings made through this effort might naturally be limited by a particular portfolio profile, they also depend on the overall structure of the firm’s business as the capital floor might cancel out the benefit.
Some of ActiveViam's clients will run both the SA and IMA, using the SA for official reporting and simulation-based models as an internal risk management tool.
Manoj Rathi: There are several considerations for banks when deciding on their approaches. Some of these include better capital allocation, and consistency in meeting desk-level quantitative requirements (PLA and backtesting requirements). They also need sufficient data for the modelling approach to withstand the regulatory requirements and avoid risk factors falling under the NMRF category. Another consideration is the need to align different data sources.
One major trade-off is the cost involved in managing the internal models and data requirements (assuming the data is available). Some have observed that, in the current framework, the level of capital savings is not worth the effort involved for a lot of big banks in applying for the IMA. That is perhaps a reason we are seeing a lot of banks that were using the IMA under the old market risk framework shifting towards implementing the SA.
David Rogers: Quantifying the benefit of the IMA accurately is a key focus for banks. They are attempting to consider the competitive advantages to be gained from enhanced modelling capabilities against the significant implementation costs. Infrastructural considerations are key, not least because the number of computations under the IMA are at least an order of magnitude higher than their Basel 2.5 equivalents. In addition, enhanced data requirements, uncertainty around the efficacy of the RFET and the PLA test, and the interplay between the various component models, add an extra layer of complexity as to the right path forward. The topic is the subject of robust debate at senior levels, given that the capital benefit over the SA is much narrower than the present regime. However, there are other factors at play, such as a firm’s reputation and the general expectation that larger banks will face regulatory pressure to adopt internal models, all of which have to be taken into consideration.
Furthermore, a lot of the benefits may not be immediately apparent without the associated analysis tools to understand how to add a layer of optimisation – desk structure, for example – that could swing the dial significantly. Ultimately, it is a decision that is firm-specific and highly dependent on the constitution of the portfolio to provide the context around any judgement.
What strategies or tools are being implemented to increase the chances of passing the PLA test?
Hany Farag: It is an uphill battle to insist on using simple risk factors that are more traditional in risk management. It seems much more achievable for banks to pass the PLA test if they align their risk factors to the front-office versions (for example, volatility surface parametrisation, interest rate curve granularity and commodity curve granularity). You basically need to shock virtually any market variable or parameter that can move from day to day. You can always opt to live with some leakage, but doing this with dozens of desks is probably not sustainable. This also applies to NMRFs. You may try to reduce their number by reducing the risk factors and allowing some error from missing risk factors, but again the model may not be sustainable in the long term.
Anastasia Polyakova: Eligibility tests help evaluate the ability of risk models to describe observed P&L volatility. ActiveViam's clients are very focused on converging models for pricing and risk. However, in certain cases, a pragmatic choice is made to run different models or different data sources. In this case, there are a few strategies that help our clients comply with PLA.
First, they can enable daily PLA controls. Even though PLA and backtesting metrics must be recalibrated and reported quarterly, our clients are equipped to run PLA daily. This strategy helps, not only to stay in compliance with the regulation but also to implement new products more rapidly, since the impact of new products and new business on risk is evaluated on day zero.
Alternatively, to help comply with PLA, our clients can identify non-performing models. If daily monitoring shows PLA test warning signs, a client can analyse the deterioration of model performance. To achieve this, customers dissect PLA and backtest metrics according to product taxonomies, models and market data characteristics to identify non-performing risk inputs. Usually, they can correlate the non-performing model with an increase in exposure to a particular product or instrument, or simply a data quality issue. Based on this analysis, banks can choose to improve some models to help pass the tests or exclude desks from the IMA.
Another strategy is for banks to develop a shared understanding. Usually, passing PLA requires close collaboration between risk, modelling and finance departments, which might view the subject from different angles. Being able to satisfy the analytics requirements of these groups within the same tool helps to develop a shared understanding.
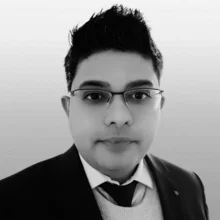
Manoj Rathi: The quality of pricing data has an important bearing on whether trading desks can pass the PLA test. Effective model validation exercises and regular model calibration have helped improve the probability of passing the test. At the same time, they have enabled banks to identify issues and address them appropriately. For example, this has helped a lot of banks identify gaps in data early on and avoid the risk of certain desks failing with the uncertainty around capital charges this brings.
David Rogers: One well-known facet of the PLA test is that it effectively asks how well P&L can be explained. Therefore, a highly directional and unbalanced portfolio is likely to perform very well on the PLA test as the drivers are well understood relative to a well-balanced and hedged portfolio. This potentially creates the unintended consequence of desks being incentivised to take additional risk and/or mandated to have a structural position that reduces the risk of – and mitigates the downside to – failing the PLA test. While this may not be the intention of the framework, the long-term solution to PLA necessitates better collaboration between risk and finance teams on P&L to explain the analysis. Many firms have systems projects in place to address deficiencies in their PLA systems, which decompose daily P&L by risk drivers.
The best of these projects target integration of both risk-based and revaluations-based attributions within a single framework, with minimal need for manual adjustments. Enhancing connectivity between PLA systems and other systems means PLA data can easily be linked to data attributes that would be required for some types of analysis, for example decomposing market-driven P&L by liquidity-related attributes, such as an International Financial Reporting Standard fair-value-levelling category, independent price verification frequency and inclusion in the risk measurement model. At this stage, firms remain in a nascent planning stage of determining what sorts of patterns and anomalies they are looking for within the PLA data, what exception reporting or tools are needed to investigate them reliably, and how P&L analysis interacts with other overlapping controls.
What strategies and data sources are being used to reduce the number of NMRFs for each trading desk?
Hany Farag: Banks are using multiple strategies and they usually need to utilise all of them. One is dimensional reduction, where banks match the dimensionality of front-office risk factors. One well-known example of this is the parametrisation of volatility surfaces. If the front office is using a specific parametrisation, risk managers may be better off mimicking that parametrisation in some form, rather than using a ‘brute force’ grid, for example. Similarly, they would want to increase the interest rate curve granularity to match that of the front office, but not any further.
The second approach is to simply buy access to vendor modellability data. The more access banks have, the more risk factors they can demonstrate as modellable. This is a costly business, and each bank is required to economise according to its activity, but will inevitably need to do something like this. Indeed, even liquid product prices may not be currently captured by the banks and, in some cases (for example, some over-the-counter products), they may have no visibility on the actual trades, even when they are numerous.
Finally, even when they cannot reduce the number of NMRFs further, they can still minimise their impact by exploiting relationships between NMRFs and modellable risk factors (MRFs). The regulations allow, if not encourage, this as it reduces the opaqueness of some risk factors arising from somewhat illiquid products.
Anastasia Polyakova: There are both pre- and post-trade processes that banks employ to help decrease the number of NMRFs, or at least minimise the impact of NMRF exposures on capital. Organisations equip trading floors with tools that help them check capital treatment of instruments and their impact on capital and other metrics.
As part of post-trade analytics, banks may choose to buy third-party observation data to increase the number of risk factors passing the RFET and source prices internally. To fine-tune the risk model, some of them may choose to bucket risk factors and proxy them with liquid inputs. This is a compromise, as it might deteriorate the model performance in the PLA tests.
Manoj Rathi: Reducing NMRFs is critical for banks to avoid adverse impacts on their capital charges and improve the accuracy of their risk modelling techniques under the IMA. Any bank that plans to implement the IMA will face this problem in some way or another at trading-desk level because of the complexity and diversity of certain instruments, such as structured products. Selecting the appropriate risk factors and ensuring completeness of data through various data sources, such as trade data, reference data and third-party providers, helps ensure modelling is accurate and meets regulatory requirements. Lots of vendors have data cleansing and validation offerings that ensure data completeness, as well as enhanced modelling techniques that help reduce the NMRFs at trading-desk level.
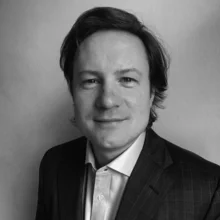
David Rogers: With regard to the NMRF framework, of crucial importance is the interplay between PLA and the RFET. The scope of NMRF risk factors is, in its most efficient and limiting cases, defined as the set of risk factors in-scope of the model that are sufficient to pass the PLA test. However, when the RFET fails, but the risk factor is necessary to pass PLA, then the NMRF needs to be capitalised. Firms must consider the available transaction information for the RFET, the likelihood of main risks in the portfolio being modellable and the full revaluation repricing for changes in the risk factors.
An important distinction to make is that the risk factor set on which we test for eligibility need not be the same as that used in valuation models. Therefore, detailed analysis is necessary to facilitate the optimal risk factor set, and may require a rethink of the set of risk factors, with a view of putting the primary risks in expected shortfall. Potential avenues being explored include making use of decompositions of NMRF to a modellable risk factor plus non-modellable basis to mitigate their impact. For credit and equity, this implies making NMRFs idiosyncratic, while for other asset classes different parametrisations can be explored. Furthermore, the rules allow firms to make use of regulatory bucketing. Within the RFET, risk factors on curves, surfaces and cubes are allowed to be bucketed together, giving a netting benefit. FRTB necessitates an extensive modelling effort to set up the tooling that generates insight into the optimal MRF and NMRF breakdown, and represents a core challenge for IMA buildouts in the coming years.
What strategies or tools are being implemented to manage changes to stress periods?
Anastasia Polyakova: There are multiple stress periods for MRFs and NMRFs, and there is a risk of jumps in capital requirements if the stress periods are calibrated every three months (as required) but have changed in between.
Stress period calibration goes together with stress-testing. If there are large market moves in line with internal stress models, as we have seen recently, this triggers the need to recalibrate the models and, at the same time, recalibrate the IMA stress periods.
What pressures is FRTB placing on banks’ data infrastructure and systems, and how are vendors helping banks navigate the remaining implementation challenges?
Hany Farag: As previously indicated, data requirements are quite high. Furthermore, the infrastructure required to support the lineage of data may be a new net improvement for banks. This is needed, for example, in the NMRF space to prove modellability and to support new risk factors.
Another new requirement imposed by FRTB is look-through for funds, which is a substantial challenge and impacts both the SA and IMA. For example, it is very difficult to obtain data for a fund that has hundreds or thousands of constituents, and even more tricky to set up each constituent in the system as if you have a position on it. Note that the constituents are not necessarily simple equity but can be bonds of various issuers, commodities, and so on. There are vendors offering various solutions from just constituent data to data with FRTB sensitivities for the SA. This alleviates some of the data challenges for the SA but certainly forces very high costs. For the IMA there does not seem to be a practical solution, and this is likely to continue to be a challenging area for several years.
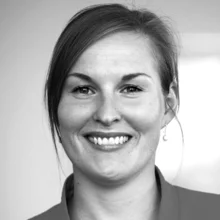
Anna Holten Møller: FRTB has been in the making for such a long time, and many banks have already implemented some of the necessary changes in data and infrastructure because other pieces of regulation have required them to do so. Generally, banks need interlinked systems for reconciliation, and they need high data quality. Vendors can assist, especially on the data side. However, increased reconciliation for regulatory reporting necessitates a centralised data pool, which probably has to be built and maintained in-house.
Anastasia Polyakova: Most of ActiveViam's clients are already in production with regard to computing official capital numbers. However, being ready to compute these numbers is not enough.
Banks now want to make the new risk infrastructure actionable. Businesses always had incentives to optimise capital utilisation. However, there was no operational readiness. Our FRTB analytics service is currently being rolled out to trading desks and risk management. This empowers individual trading desks to understand the contribution of their trading activity to the firms' capital and evaluate the impact of new trading decisions. The design of the Atoti FRTB solution is driven by the requirement to flexibly identify contributors, evaluate allocations and potentially use portfolio scenarios for capital utilisation.
At the same time, middle- and back-office teams are preparing to run market risk production processes daily. With the traditional market risk methodology, chief risk officers had a dream of completing risk processes on T0, with the reality of T+1 deadlines. With the new methodology, certain teams take weeks to complete FRTB numbers. Today, there is an opportunity to review processes and infrastructure to ensure daily production is streamlined and T1 (or even T0) is possible in the future. As banks continue to re-evaluate data sources, and upgrade individual pricing and risk components, we build tools to help users investigate and validate data quickly, adjust and sign-off. They can then publish official risk results together with the governance of meta information in data warehouses in a streamlined fashion.
Manoj Rathi: The primary challenges banks face concern the data requirements and IT infrastructure to handle the complex regulatory calculations efficiently, irrespective of whether they opt for the SA or IMA. Vendors are ensuring the data requirements are standardised and reusability is improved. There are other important implementation challenges for banks operating in multiple jurisdictions with different versions of FRTB. Vendors are playing a crucial role in ensuring local variations are incorporated rapidly and banks can avoid the need to source subject matter experts across jurisdictions. This allows banks to focus more on portfolio construction and optimising market risk capital charges. By offering granular-level data analysis from risk factor input to final reporting, vendors are helping banks improve transparency and meet the reporting requirements.
The panellists’ responses to our questionnaire were made in a personal capacity, and the views expressed herein do not necessarily reflect or represent the views of their employing institutions.
Sponsored content
Copyright Infopro Digital Limited. All rights reserved.
As outlined in our terms and conditions, https://www.infopro-digital.com/terms-and-conditions/subscriptions/ (point 2.4), printing is limited to a single copy.
If you would like to purchase additional rights please email info@risk.net
Copyright Infopro Digital Limited. All rights reserved.
You may share this content using our article tools. As outlined in our terms and conditions, https://www.infopro-digital.com/terms-and-conditions/subscriptions/ (clause 2.4), an Authorised User may only make one copy of the materials for their own personal use. You must also comply with the restrictions in clause 2.5.
If you would like to purchase additional rights please email info@risk.net