
Quant of the year: Alexei Kondratyev
Risk Awards 2019: A glimpse of the future? Quant uses ML to model term structure and crunch margin costs
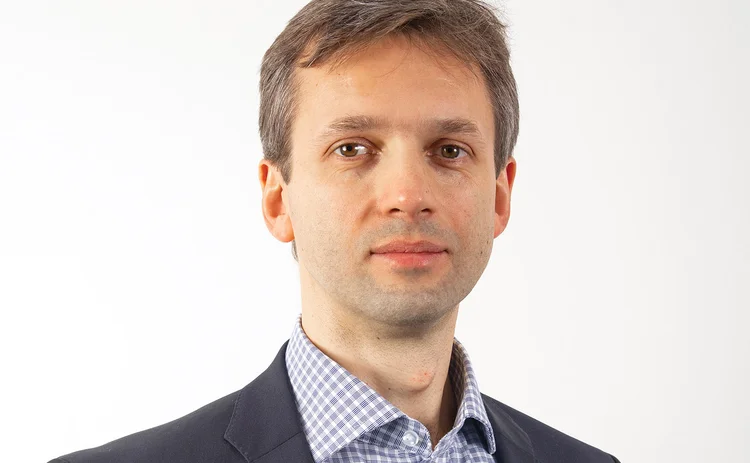
Ten years ago, if quants were told to discard their prized stochastic models and let machines predict the movement of assets even if, in some cases, they couldn’t fully comprehend how the machine does this, they would have been dismayed or disbelieving. Or both.
Today, the ability to adapt to such a change – even to facilitate it – may be a prerequisite for survival as a quant.
Alexei Kondratyev, the head of the recently created data analytics group at Standard Chartered, has proved this in more than one way over the past year, managing to attract some fans along the way.
“I think it’s really refreshing to see someone who can work in multiple areas,” says Alexander Sokol, the chief executive of vendor CompatibL. “Quants these days tend to maintain expertise in specific fields. With Alexei, his expertise in multiple, unrelated fields gives him a broader perspective and makes him a great researcher.”
This is evident in Kondratyev’s work. After spending most of his career in risk teams, he is now helping explore the application of powerful new technologies to front-office problems in finance.
Machine learning – which uses algorithms to perform tasks based on what it learns from an underlying dataset – has found one of its most common front-office uses on the buy side, among firms trying to understand the market impact of big trades. Uptake on the sell side has been slower.
Through two recent papers published on Risk.net, Kondratyev has opened up the possibility of more widespread applications for machine learning on the sell side, specifically focusing on the optimisation of margin costs and the building of interest rate curves.
The first of these techniques has helped StanChart reduce the initial margin requirements for the non-cleared trades to which it has been applied by up to half.
“Once we saw how powerful these tools can be, we started to look at different machine learning tools, techniques and use cases, and it opened so many opportunities for us,” says Kondratyev. “You taste it once – you can’t forget this taste – you want more.”
What started in 2015 as a personal project for Kondratyev to explore the uses of machine learning has now generated enough excitement within StanChart that the bank created a standalone data analytics group in June this year.
The group, which Kondratyev heads, has a mandate to generate revenues across the bank through the use of new technologies, including still-unproven areas of research such as quantum computing.
“We decided we have to apply machine learning tools systematically and look at data systematically, and this is the way for us to differentiate ourselves from our competition,” says Kondratyev.
Sell-side problem
The more popular of Kondratyev’s two papers published on Risk.net last year is Curve dynamics with artificial neural networks, in which he uses a machine learning technique called artificial neural networks to build interest rate curves.
Capturing term structure dynamics has been a notoriously non-linear problem on the sell side due to the spread of near-zero and negative interest rates in the decade since the financial crisis. Around these levels, rates behave differently, and classical models were not equipped to cope.
The industry’s standard approach to term structure modelling has been to use principal component analysis (PCA), which tries to reduce a large set of variables describing a dataset – it does that by decomposing a covariance matrix of correlated variables into a set of uncorrelated ones, called principal components.
The trouble with the PCA is that it relies mainly on a measure of linear relationship – correlation. So a lot of information about non-linear relationships is ignored.
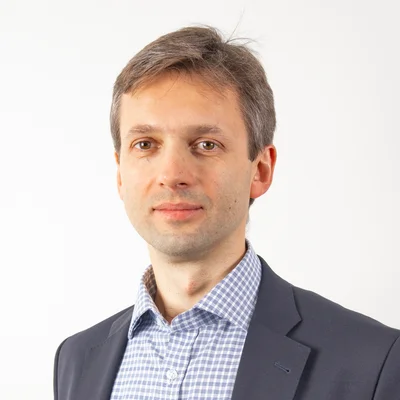
“Say, the curve is specified on 10 tenors and we need to construct a 10-by-10 covariance matrix. We might have daily observations for the past 25 years but everything is compressed into just 100 values,” says Kondratyev.
In his paper, Kondratyev applies an artificial neural network to solve this problem. Such a network is made up of thousands of connections between hundreds of nodes that can take in the input dataset – used to train the algorithm – and through many iterations of non-linear regressions estimate the coefficients or variables that drive, in this example, the rates.
Because there are a large number of coefficients describing the behaviour of rates, the quant forces some coefficients to be zero or to take on small values – a technique called regularisation – to eliminate overfitting.
One particularly interesting feature of Kondratyev’s approach is his use of an auto-encoder, an artificial neural network that tries to represent a large input dataset using lower dimensions, or a smaller number of variables. The idea is to get a sense of whether the current input curve is abnormal, by comparing it with the simplified smoother curve produced by the auto-encoder.
The auto-encoder can also suggest a way to correct a distorted curve. At each iteration step, the network propagates the error between model output and actual output based on the data backwards, so coefficients can be adjusted to better reflect actual dynamics.
Other quants agree auto-encoders are a good fit for the job.
“Anything that is non-linear, which is one level higher in terms of complexity within the dynamics, will not be captured by PCA. Something like the auto-encoder will allow you to do that – it is a very good tool to do that,” says Youssef Elouerkhaoui, a managing director and quant at Citi in London.
Learning machine learning
After completing his PhD in physics in 1997 from the National Academy of Sciences of Ukraine, Kondratyev started his career in finance as an equity analyst at an American brokerage in Kiev. That role ended with the Russian debt default in 1998, spurring Kondratyev to become what he calls a “proper” quant at the Center of Advanced European Studies and Research in Bonn.
In 2001, he joined the risk function at Dresdner Bank, followed by similar roles at Barclays in 2004 and StanChart in 2010. He helped build risk engines from scratch at all three banks.
In 2013, Kondratyev made an unusual move from the risk function to the front office at StanChart, where he was asked to build a bilateral trade compressor to help reduce the bank’s risk-weighted assets (RWA) as part of a broader move by large dealers at the time to optimise their balance sheets.
The bank achieved an $8.5 billion RWA reduction from 2013 to 2014. It is understood around a quarter of this number came through bilateral trade compression.
It was during this period that Kondratyev started to lay the foundations for the first derivatives valuation adjustment desk – or XVA desk – at StanChart, such as netting and collateral set construction tools.
Until the end of 2015, the bank had been using an older method when calculating credit valuation adjustment (CVA), producing lower CVA charges. In 2015, Bill Winters became the group chief executive, bringing discipline learned during his time running JP Morgan’s investment bank, and StanChart swiftly adopted a more rigorous industry standard for CVA calculation. The result was a one-off loss of $712 million, as the bank remarked its books.
“We only created a proper XVA desk when Bill Winters joined the bank in 2015. From 2015 onwards, we had a discipline behind recognising funding valuation adjustment and CVA,” says Kondratyev.
It was his experience in building the analytics tools for the XVA desk and his subsequent role heading the bank’s prime services analytics group from 2015 to 2018 that led Kondratyev to look at the use of machine learning for portfolio optimisation.
“Being head of analytics at prime services, part of my task was to look at portfolio optimisation. So we already spent so much time looking at machine learning,” says Kondratyev.
Sowing the seeds
Once new margining rules for non-cleared derivatives went live in September 2016, Kondratyev started working on his first paper on machine learning, Evolutionary algos for optimising MVA.
In an attempt to replicate one of the benefits of central clearing, margin rules require derivatives users to post initial margin (IM) on non-cleared trades with other in-scope counterparties based on the standard initial margin model (Simm) created by the industry. This IM has to be funded and the cost should in theory be priced into trades in the form of a margin valuation adjustment (MVA), which is becoming increasingly material.
“We didn’t expect Simm IM to start growing so fast and reaching such a big amount so quickly, so we started looking at ways to optimise it,” says Kondratyev.
By applying two machine learning algorithms, which belong to the class of so-called evolutionary algorithms, Kondratyev and his co-author George Giorgidze – a senior quantitative developer at StanChart – devised a way to reduce margin costs over the life of the portfolio, while keeping the market risk exposure the same.
Evolutionary algorithms run multiple iterations of chromosomes, tweaking one or two genes at a time, to find the most beneficial mutation. In this way, the algorithms work like evolution, by randomly generating trades – the genes – by tweaking one or two trade details such as direction, notional size and currency. They keep mutations that reduce MVA and discard those that increase it.
The technique has been in use at StanChart since 2017, helping the bank reduce Simm IM by up to half where applied, Kondratyev says.
This initial success sowed the seeds for broader application of the tool at StanChart, mandated by senior management.
Quantum leap
What’s next for Kondratyev? The quant says he will be exploring more use cases for machine learning as part of his new role as the head of the data analytics group at StanChart. There will also be a focus on quantum computing.
Quantum computing uses certain properties of particles to carry out computations more rapidly than classical computers and is already being explored by banks such as Barclays and JP Morgan. Some groundwork has been done at StanChart as well.
At the start of 2018, Kondratyev led collaboration with space agency Nasa and the Universities Space Research Association in the US to apply quantum computing to portfolio optimisation. A quantum computer took half the time of a classical computer to optimise a 60-asset portfolio.
While critics say it will take a while for the technology to take off in finance due to its vulnerability to errors, Kondratyev remains optimistic.
“I believe it will transform not only finance but also everything around us… My personal prediction, which is perhaps slightly on the optimistic side, is that we are three to five years away from using quantum computing for real-world applications,” he says.
Only users who have a paid subscription or are part of a corporate subscription are able to print or copy content.
To access these options, along with all other subscription benefits, please contact info@risk.net or view our subscription options here: http://subscriptions.risk.net/subscribe
You are currently unable to print this content. Please contact info@risk.net to find out more.
You are currently unable to copy this content. Please contact info@risk.net to find out more.
Copyright Infopro Digital Limited. All rights reserved.
As outlined in our terms and conditions, https://www.infopro-digital.com/terms-and-conditions/subscriptions/ (point 2.4), printing is limited to a single copy.
If you would like to purchase additional rights please email info@risk.net
Copyright Infopro Digital Limited. All rights reserved.
You may share this content using our article tools. As outlined in our terms and conditions, https://www.infopro-digital.com/terms-and-conditions/subscriptions/ (clause 2.4), an Authorised User may only make one copy of the materials for their own personal use. You must also comply with the restrictions in clause 2.5.
If you would like to purchase additional rights please email info@risk.net
More on Awards
Environmental products house of the year: ENGIE
ENGIE is driving change in energy transition, with a strong focus on renewable energy and the liberalisation of power markets in Apac, which presents significant long-term growth opportunities. In recognition of its efforts, ENGIE GEMS has been named…
Natural gas/LNG house of the year: ENGIE
ENGIE continues to expand its services to better serve firms in Apac dealing with the challenges of energy risk management and supply
FRTB management solution of the year: Bloomberg
Amid the diverging timeframes and complex requirements of FRTB, Bloomberg offers a consistent, comprehensive and customisable solution for Apac banks preparing for implementation
Newcomer of the year: Topaz Technology
Jon Fox and former colleagues formed Topaz Technology in 2015. Having seen many different systems and, in some cases, written and built a few themselves, there was always something missing, leading them to build a system that unifies risk reporting and…
Technology vendor of the year: Murex
As a technology vendor, Murex places adaptability front and centre of everything it does, constantly enriching its MX.3 platform to ensure institutions can respond to new market opportunities as soon as they spot them
Currency derivatives house of the year: Deutsche Bank
Asia Risk Awards 2024
Interest rate derivatives house of the year: Standard Chartered Bank
Asia Risk Awards 2024
Derivatives house of the year, Taiwan: CTBC Bank
Asia Risk Awards 2024